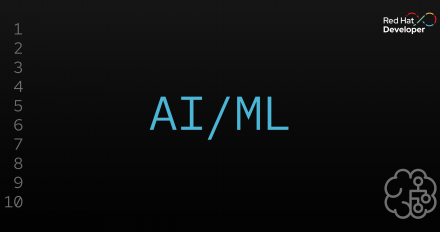
Compressed Granite 3.1: Powerful performance in a small package
Open-sourced on Hugging Face, deployment-ready with vLLM, and extensible using LLM Compressor.
Open-sourced on Hugging Face, deployment-ready with vLLM, and extensible using LLM Compressor.
Let's take a look at how to effectively integrate Generative AI into an existing application through the InstructLab project, an open-source methodology and community to make LLM tuning accessible to all! Learn about the project, and how InstructLab can help to train a model on domain-specific skills and knowledge, then how Podman's AI Lab allows developers to easily setup an environment for model serving and AI-enabled application development.
The Konveyor community has developed "Konveyor AI" (Kai), a tool that uses Generative AI to accelerate application modernization. Kai integrates large language models with static code analysis to facilitate code modifications within a developer's IDE, helping transition to technologies like Quarkus efficiently. This video provides a short introduction and demo showcasing the migration of the Java EE "coolstore" application to Quarkus using Konveyor AI.
In this episode, Senior Distinguished Engineer Dan Walsh discusses tips and tricks for writing SELinux policies and how you can use containers to your advantage.
Welcome to the new Red Hat Dan on Tech, where Senior Distinguished Engineer Dan Walsh dives deep on all things technical, from his expertise in container technologies with tools like Podman and Buildah, to runtimes, Kubernetes, AI, and SELinux! This weekly series will bring in guests from around the industry to highlight innovation and things you should know, and new episodes will be released right here, on the Red Hat Developer channel, each and every Wednesday at 9am EST! Stay tuned, and see you in the next episode!
Kickstart your generative AI application development journey with Podman AI Lab, an open-source extension for Podman Desktop to build applications with LLMs on a local environment. The Podman AI Lab helps to make AI more accessible and approachable, providing recipes for example use cases with generative AI, curated models sourced from Hugging Face, model serving with integrated code snippets, and a playground environment to test and adjust model performance. Learn more on Red Hat Developer https://developers.redhat.com/product... and download Podman Desktop today to get started!
Let's take a look at how you can get started working with generative AI in your application development process using open-source tools like Podman AI Lab (https://podman-desktop.io/extensions/...) to help build and serve applications with LLMs, InstructLab (https://instructlab.ai) to fine-tune models locally from your machine, and OpenShift AI (https://developers.redhat.com/product...) to handle the operationalizing of building and serving AI on an OpenShift cluster.
Learn about the alpha release of vLLM V1, a major upgrade to vLLM’s core architecture.
Model Context Protocol (MCP) is a protocol that allows intergratrion between
Advancing AI efficiency is more critical than ever, and sparsity has proven to be a cornerstone in this pursuit.
Quantized LLMs achieve near-full accuracy with minimal trade-offs after 500K+ evaluations, providing efficient, high-performance solutions for AI model deployment.
Machete, Neural Magic’s optimized kernel for NVIDIA Hopper GPUs, achieves 4x memory savings and faster LLM inference with mixed-input quantization in vLLM.
Discover LLM Compressor, a unified library for creating accurate compressed models for cheaper and faster inference with vLLM.
Explore the integration of FP8 in vLLM. Learn how to receive up to a 2x reduction in latency on NVIDIA GPUs with minimal accuracy degradation.
Llama 3's advancements, particularly at 8 billion parameters, make AI more accessible and efficient.
Learn about Marlin, a mixed-precision matrix multiplication kernel that delivers 4x speedup with FP16xINT4 computations for batch sizes up to 32.
4-bit and 8-bit quantized LLMs excel in long-context tasks, retaining over 99% accuracy across 4K to 64K sequence lengths.
Sparse fine-tuning in combination with sparsity-aware inference software, like DeepSparse, unlocks ubiquitous CPU hardware as a deployment target for LLM inference.
Compress large language models (LLMs) with SparseGPT to make your machine learning inference fast and efficient. Prune in one-shot with minimal accuracy loss.
Gather the data you collect into real-time information you can use to optimize