Page
Prerequisites and step-by-step guide
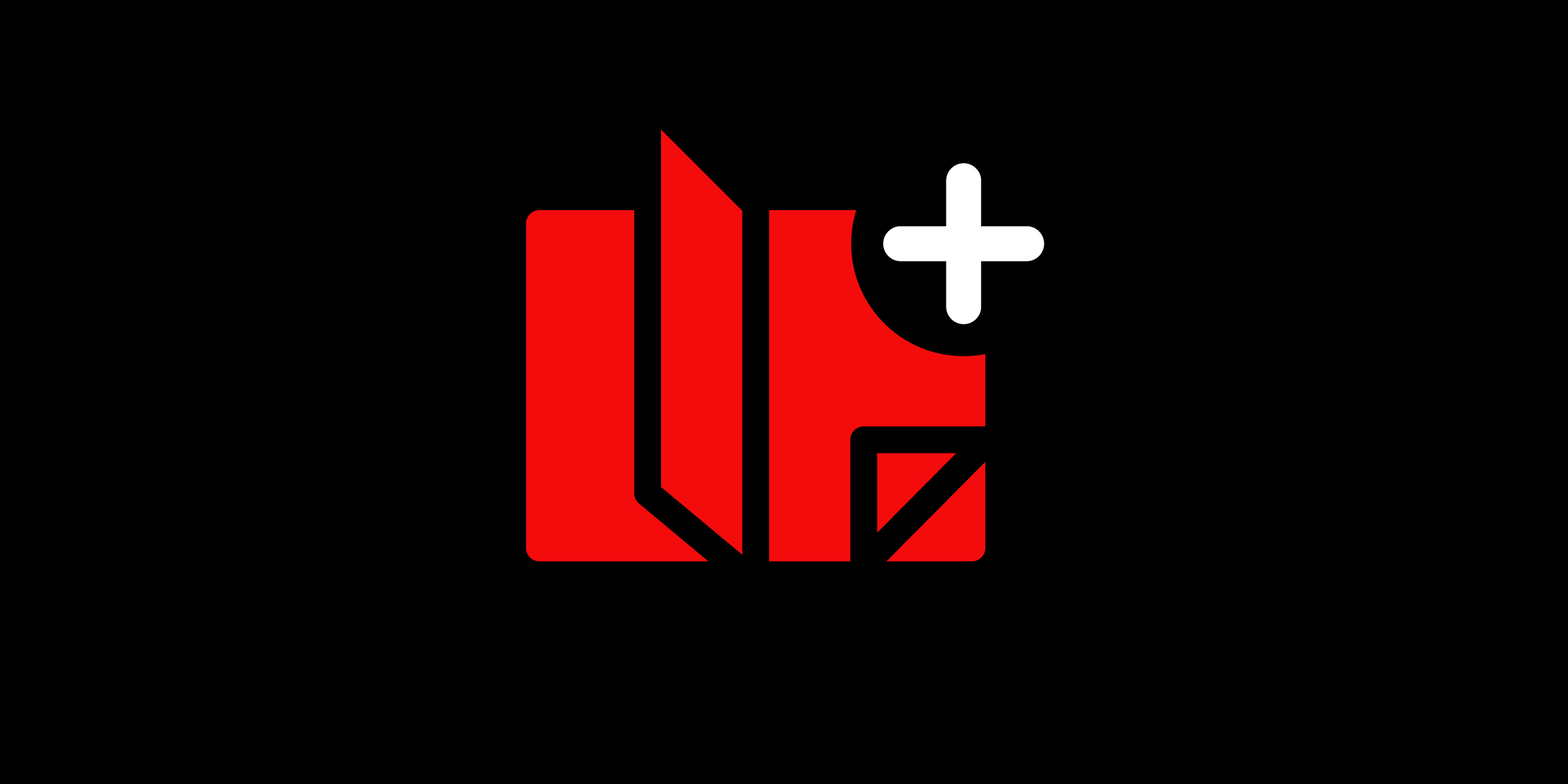
Prerequisites
To run through the learning exercise you need to install Node.js and to clone the ai-experimentation repository. To set up these prerequisites:
- If you don’t already have Node.js installed, install it using one of the methods outlined on the Nodejs.org download page.
Clone the ai-experimentation repository with:
git clone https://github.com/mhdawson/ai-experimentation
- cd ai-experimentation/lesson-3-4
- Create a directory called models.
- Download the mistral-7b-instruct-v0.1.Q5_K_M.gguf model from HuggingFace and put it into the model’s directory. This might take a few minutes, as the model is over 5GB in size.
Install the applications dependencies with
npm install
Copy over the markdown files for the Node.js Reference Architecture into the directory from which we’ll read additional documents to be used for context:
mkdir SOURCE_DOCUMENTS git clone https://github.com/nodeshift/nodejs-reference-architecture.git cp -R nodejs-reference-architecture/docs SOURCE_DOCUMENTS
For windows use the file manager and make sure to copy the docs and all subdirectories to SOURCE_DOCUMENTS.
Step-by-step guide
1. Exploring the code in langchainjs-rag.mjs.
The code in langchainjs-rag.mjs code starts by loading the markdown files from the Node.js reference architecture into an in-memory vector database which is available in Langchain.js:
////////////////////////////////
// LOAD AUGMENTING DATA
// typically this is stored in a database versus being loaded every time
console.log("Loading and processing augmenting data - " + new Date());
const docLoader = new DirectoryLoader(
"./SOURCE_DOCUMENTS",
{
".md": (path) => new TextLoader(path),
}
);
const docs = await docLoader.load();
const splitter = await new MarkdownTextSplitter({
chunkSize: 500,
chunkOverlap: 50
});
const splitDocs = await splitter.splitDocuments(docs);
const vectorStore = await MemoryVectorStore.fromDocuments(
splitDocs,
new HuggingFaceTransformersEmbeddings()
);
const retriever = await vectorStore.asRetriever();
console.log("Augmenting data loaded - " + new Date());
The first part uses the DirectoryLoader API to recursively load all of the documents we copied in the SOURCE_DOCUMENTS directory. For each markdown file ending in “.md” it uses the TextLoader API to load the document. There is built in support for a number of different document types including CSV, JSON, PDF and more. You can read about these in the Document Loaders section of the Langchain.js documentation.
Once loaded, the documents need to be split into chunks that can be indexed and retrieved in order to provide additional context to queries made to the model. To do this we use the MarkdownTextSplitter API to break the documents up into chunks of 500 bytes.
Once the documents are split into chunks, the MemoryVectorStore API is used to create an in-memory database for the split documents using the HuggingFaceTransformersEmbeddings API as the embeddings used to create the set of vectors used to index each chunk. There are a number of different embeddings supported by Langchain.js and the one most appropriate may depend on the model being used. In our case the HuggingFaceTransformersEmbeddings seemed to be effective.
Finally we get an instance of the Retriever API that can be used to lookup chunks based on the query.
In a real application we would not read the documents every time and instead use a persistent database. For this example loading the documents every time seems to take about 10-20 seconds which is a reasonable tradeoff for keeping the example simple.
Using the retriever created we can find chunks matching the question used in our examples with:
retriever.getRelevantDocuments(“Should I use npm to start a node.js application?");
We’ll see later on in the exercise the documents returned for that query.
One we have the documents ingested was can use the LangChain expression language to compose a chain that will use the ingested data when asking our question:
////////////////////////////////
// CREATE CHAIN
const prompt =
ChatPromptTemplate.fromTemplate(`Answer the following question based only on the provided context, if you don't know the answer say so:
<context>
{context}
</context>
Question: {input}`);
const documentChain = await createStuffDocumentsChain({
llm: model,
prompt,
});
const retrievalChain = await createRetrievalChain({
combineDocsChain: documentChain,
retriever,
});
The createStuffDocumentsChain takes a list of documents, formats them into a prompt and sends it on to the model. We phrase the prompt in a way which frames the question and includes the documents which are passed in the {context} key.
The retrievalChain takes the query to be sent to the model along with a retriever that will lookup related document chunks using the retriever passed in. We pass it the StuffDocumentsChain which will then use the document chunks to format the full prompt sent to the model.
You can read more about chains and how to compose them in the chains section of the Langchain.js documentation.
Now that we’ve built the chain we can ask it our question:
////////////////////////////////
// ASK QUESTIONS
console.log(new Date());
let result = await retrievalChain.invoke({
input: "Should I use npm to start a node.js application",
});
console.log(result);
console.log(new Date());
2. Running the RAG Langchain.js example
You can run the RAG example with (this will take a minute or two if you are not on a platform where GPU acceleration is available/supported):
node langchainjs-rag.mjs
The answer may vary, but you should get an answer which reflects the recommendations in the Node.js Reference Architecture:
'Assistant: It is generally not necessary to use `npm` to start a Node.js application. If you avoid using it in the container, you will not be exposed to any security vulnerabilities that might exist in that component or its dependencies. However, it is important to build security into your software development process when developing Node.js modules and applications. This includes managing dependencies, managing access and content of public and private data stores such as npm and github, writing defensive code, limiting required execution privileges, supporting logging and monitoring, and externalizing secrets.'
Looking at the output we can see that in addition to the answer we’ve printed the document chunks that were included in the context sent to the model. As mentioned before, the total size of the prompt including the context is limited. The retriever helps us select the document chucks most relevant to the question and include them in the context.
context: [
Document {
pageContent: '## avoiding using `npm` to start application\r\n' +
'\r\n' +
'While you will often see `CMD ["npm", "start"]` in docker files\r\n' +
'used to build Node.js applications there are a number\r\n' +
'of good reasons to avoid this:\r\n' +
'\r\n' +
"- One less component. You generally don't need `npm` to start\r\n" +
' your application. If you avoid using it in the container\r\n' +
' then you will not be exposed to any security vulnerabilities\r\n' +
' that might exist in that component or its dependencies.',
metadata: [Object]
},
Document {
pageContent: '* [Introduction to the Node.js reference architecture: Node Module Development](https://developers.redhat.com/articles/2023/02/22/installing-nodejs-modules-using-npm-registry)',
metadata: [Object]
},
Document {
pageContent: '# Secure Development Process\r\n' +
'\r\n' +
'It is important to build security into your software development process. Some of the key elements to address in the development process for Node.js modules and applications include:\r\n' +
'\r\n' +
'* Managing dependencies\r\n' +
'* Managing access and content of public and private data stores\r\n' +
' such as npm and github \r\n' +
'* Writing defensive code\r\n' +
'* Limiting required execution privileges\r\n' +
'* Supporting logging and monitoring\r\n' +
'* Externalizing secrets',
metadata: [Object]
},
Document {
pageContent: '## Further Reading\r\n' +
'\r\n' +
'* [Introduction to the Node.js reference architecture: Node Module Development](https://developers.redhat.com/articles/2023/02/22/installing-nodejs-modules-using-npm-registry)\r\n' +
'\r\n' +
'* https://github.blog/changelog/2020-10-02-npm-automation-tokens/\r\n' +
'\r\n' +
'* https://medium.com/@alex.birsan/dependency-confusion-4a5d60fec610',
metadata: [Object]
}
],
Looking at the answer and the context you can see that the answer is based in part on the matching document chunks.
If you want to see what the answer looks like without the information added through Retrieval Augmented Generation, you can delete the files under the SOURCE_DOCUMENTS
directory and run the application again. If you do that you’ll see the answer that the large language model would have returned without the information in the Node.js Reference Architecture.
In this learning exercise we demonstrated using Retrieval Augmented Generation with LangChain.js. We introduced the APIs for document loading, splitting and retrieval along with the APIs the StuffDocumentsChain and RetrievalChain APIs using the LangChain expression Language to compose together.