“Attention is all you need.” But you also need efficient GPU peer-to-peer communication!
Since that seminal paper was published in 2017, scaling the size of large language models (LLMs) has been a key driver to sustain the pace of innovation and push the frontier of LLM performance while informing the so-called scaling laws of AI.
While techniques like quantization or compression can be used to lower the amount of memory required to load those large models into a single GPU, it comes with some tradeoffs, and only shifts the threshold beyond which scaling out becomes the only viable option. In the case of model training, scaling-out can also be used to leverage more compute resources and achieve better latency.
Communication overhead
Scaling out deep learning, however, induces a significant communication overhead between the GPUs to carry the computation. For example, when training models using PyTorch FSDP or DeepSpeed ZeRO, sharded weights are gathered on all GPUs before every layer forward and backward passes, and local gradients are reduced and scattered at the end of every mini-batch. Depending on the size of the model and the number of GPUs, this can represent a peak traffic of multiple Gbit/s.
In a standard OpenShift cluster, that traffic transits via the default OVN-Kubernetes network CNI plugin. It relies on the Open Virtual Network (OVN), a network virtualization solution, that provides general-purpose pod-to-pod communication that’s not tailored for AI applications. In practice it proves to slow significantly down the distributed model training to the point where it becomes the bottleneck as illustrated in Fine-tune LLMs with Kubeflow Trainer on OpenShift AI.
Companies like Meta and IBM have spearheaded training open source LLMs and shared their research on infrastructure design to alleviate that bottleneck. Be it in RDMA over Ethernet for Distributed AI Training at Meta Scale or The infrastructure powering IBM’s Gen AI model development, both companies have highlighted how critical low-latency / high bandwidth networking is to efficiently scale distributed model training and choose to use GPUDirect RDMA over Converged Ethernet (RoCE) to train their Llama or Granite models.
Starting with Red Hat OpenShift AI 2.19, you can leverage networking platforms such as NVIDIA Spectrum-X with high-speed GPU interconnects to accelerate model training using GPUDirect RDMA over Ethernet or InfiniBand physical link.
After a technical overview of the solution, this article demonstrates how to adapt the example from Fine-tune LLMs with Kubeflow Trainer on OpenShift AI so it runs on Red Hat OpenShift Container Platform with accelerated NVIDIA networking and gives you a sense of how it can improve performance dramatically!
Solution overview
NVIDIA and Red Hat have partnered for many years to bring the best of NVIDIA accelerated computing and networking stack and Red Hat’s leading container orchestration platform, OpenShift. Thanks to this collaboration and many communities powering a global open source ecosystem, Red Hat OpenShift AI can layer on top of this reference architecture (Figure 1) for high performance distributed model training.
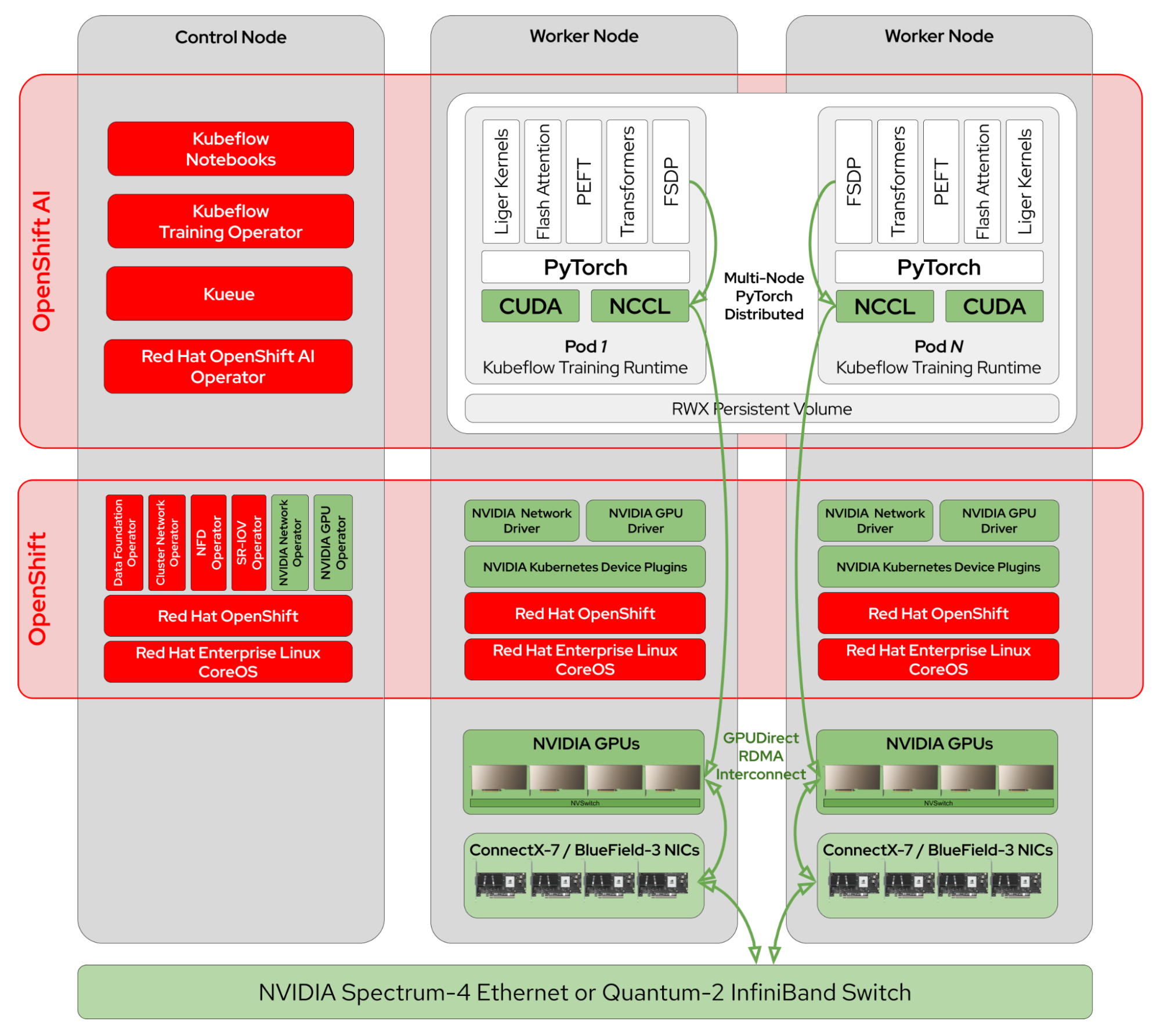
This solution includes in particular:
- The NVIDIA Network Operator that automates the deployment of NVIDIA networking components on the nodes like networking drivers, device plugins, secondary network NIC plugins and NVIDIA NIC Feature Discovery.
- The NVIDIA GPU Operator that automates the deployment of software components on the nodes so containers can use GPUs and works with the NVIDIA Network Operator to enable GPUDirect RDMA between GPUs and NICs.
- NVIDIA Collective Communication Library (NCCL) that implements collective operations and detects the GPU interconnects topology to optimize intra-node and inter-node communication using NVLink and GPUDirect RDMA when available, and that’s integrated in PyTorch Distributed as communication back end.
- PyTorch Fully Sharding Data Parallel (FSDP) that shards model across workers and distributes the training so it’s possible to train very large models on multiple GPUs.
- The HuggingFace Transformers and Parameter-Efficient Fine-Tuning (PEFT) libraries that abstract all the complexity to handle pre-trained models and implement Supervised Fine-Tuning (SFT) as well as Low-Rank Adaptation (LoRA).
- The Kubeflow Training Operator that configures PyTorch distributed training jobs on OpenShift.
- Kueue, which provides GPUs quota management and fair-sharing usage in multi-tenant environments.
- The OpenShift Cluster Network Operator that configures the OVN-Kubernetes CNI plugin (by default) and deploys the Multus CNI plugin that manages the attachment of secondary network interfaces to pods.
- The SR-IOV Operator that sets up the SR-IOV stack so it’s possible to attach compatible PCIe network devices to multiple pods via virtual functions.
- The OpenShift Data Foundation Operator, that provides shared filesystem volumes provisioning used for distributed checkpointing.
LLM fine-tuning example
The previous article Fine-tune LLMs with Kubeflow Trainer on OpenShift AI demonstrates how to fine-tune the Llama 3.1 8B Instruct model on the GSM8K dataset. The “Observe and experiment” section of the article highlights how much peer-to-peer GPU traffic is generated during fine-tuning and hypothetizes the default OpenShift Open Virtual Network (OVN) network could be a performance bottleneck.
The following sections aim at validating that hypothesis. First, we'll describe how we’ve configured OpenShift Container Platform to enable GPUDirect RDMA over Ethernet (RoCE) with NVIDIA Spectrum-X network platform hardware components such as the Spectrum-4 SN5600 Ethernet switch and BlueField-3 SuperNICs. Then we'll show how to adapt the fine-tuning example from the previous article so it leverages this accelerated network configuration and finally compares the performance.
Configure the networking platform
There are multiple supported configurations to enable NVIDIA networking platforms; it's beyond the scope of this article to go over all of them. We recommend the Getting Started with Red Hat OpenShift part of the NVIDIA Network Operator documentation that covers deployment examples for OpenShift Container Platform in detail as well as the GPUDirect RDMA section of the NVIDIA GPU Operator documentation in particular.
The performance results we present in the “Compare Performance” section below have been produced using the Network Operator deployment with RDMA Shared Device Plugin and we only highlight here the information that are relevant for configuring the LLM fine-tuning example in the next “Run the LLM Fine-Tuning Example” section.
The NicClusterPolicy
resource with the extended resource names in the rdmaSharedDevicePlugin
field corresponding to the RoCE network interfaces that are going to be used in the fine-tuning job resource requests and limits:
apiVersion: mellanox.com/v1alpha1
kind: NicClusterPolicy
metadata:
name: nic-cluster-policy
spec:
nicFeatureDiscovery:
image: nic-feature-discovery
repository: ghcr.io/mellanox
version: v0.0.1
nvIpam:
image: nvidia-k8s-ipam
repository: ghcr.io/mellanox
version: v0.2.0
ofedDriver:
repository: nvcr.io/nvidia/mellanox
image: doca-driver
rdmaSharedDevicePlugin:
config: |
{
"configList": [
{
"resourceName": "rdma_shared_device_eth",
"rdmaHcaMax": 63,
"selectors": {
"ifNames": ["ens8f0np0"]
}
}
]
}
repository: ghcr.io/mellanox
version: v1.5.2
The MacvlanNetwork
resource for RDMA over Converged Ethernet (RoCE):
apiVersion: mellanox.com/v1alpha1
kind: MacvlanNetwork
metadata:
name: rdmashared-net
spec:
ipam: '{"type": "whereabouts", "range": "192.168.2.0/24", "gateway": "192.168.2.1"}'
master: ens8f0np0
mode: bridge
mtu: 1500
The generated NetworkAttachementDefinition
, which will be referred to later in the fine-tuning job:
apiVersion: k8s.cni.cncf.io/v1
kind: NetworkAttachmentDefinition
metadata:
name: rdmashared-net
spec:
config: '{ "cniVersion":"0.3.1", "name":"rdmashared-net", "type":"macvlan","master": "ens8f0np0","mode" : "bridge","mtu" : 1500,"ipam":{"type":"whereabouts","range":"192.168.2.0/24","gateway":"192.168.2.1"} }'
Finally, the ClusterPolicy
that configures the NVIDIA GPU Operator should be updated to enable GPUDirect RDMA on GPU devices (excerpted):
apiVersion: nvidia.com/v1
kind: ClusterPolicy
metadata:
name: gpu-cluster-policy
spec:
driver:
rdma:
enabled: true
Configure the container engine (CRI-O) to enable RDMA for non-root users
For the inter-node communication between GPUs to happen over RDMA and without buffer copies, pinned memory blocks on host memory filled with virtual addresses need to be allocated and registered via CUDA. The amount of memory that can be allocated for non-root users is usually restricted to a default value that’s too limited for what NCCL typically needs.
To be able to run the training process as a non-root user and with the most restrictive OpenShift default security context, this limit has to be increased in the container engine (CRI-O) configuration. This can be achieved with the Machine Configuration Operator (MCO), for example:
apiVersion: machineconfiguration.openshift.io/v1
kind: MachineConfig
metadata:
labels:
machineconfiguration.openshift.io/role: worker
name: 02-worker-container-runtime
spec:
config:
ignition:
version: 3.2.0
storage:
files:
- contents:
inline: |
[crio.runtime]
default_ulimits = [
"memlock=-1:-1"
]
mode: 420
overwrite: true
path: /etc/crio/crio.conf.d/10-custom
This example enables an unlimited amount of pinned memory to be allocated by userspace applications, but it’s recommended you set it to a fixed limit large enough for your applications instead. Once the MachineConfig resource is created, the worker nodes must be restarted for it to be taken into account.
Run the LLM fine-tuning example
In the article Fine-tune LLMs with Kubeflow Trainer on OpenShift AI, we used the KubeFlow SDK to configure and create the distributed PyTorch job. In this article, we show you how to use the PyTorchJob API directly, and highlight the few changes needed so it leverages the networking configuration described in the previous section. This way, you can understand the relationship between the two and be able to apply it on your own accelerated networking platform.
First, create the following configuration file named config.yaml
that contains the parameters to fine-tune the Llama 3.1 8B Instruct pre-trained model using the GSM8K dataset from Hugging Face:
# Model
model_name_or_path: Meta-Llama/Meta-Llama-3.1-8B-Instruct
model_revision: main
torch_dtype: bfloat16
attn_implementation: flash_attention_2 # one of eager (default), sdpa or flash_attention_2
use_liger: true # use Liger kernels
# PEFT / LoRA
use_peft: true
lora_r: 16
lora_alpha: 8
lora_dropout: 0.05
lora_target_modules: ["q_proj", "v_proj", "k_proj", "o_proj", "gate_proj", "up_proj", "down_proj"]
# Dataset
dataset_name: gsm8k # id or path to the dataset
dataset_config: main # name of the dataset configuration
dataset_train_split: train # dataset split to use for training
dataset_test_split: test # dataset split to use for evaluation
dataset_text_field: text # name of the text field of the dataset
# SFT
max_seq_length: 4096 # max sequence length for model and packing of the dataset
# Training
num_train_epochs: 10 # number of training epochs
per_device_train_batch_size: 32 # batch size per device during training
per_device_eval_batch_size: 32 # batch size for evaluation
eval_strategy: epoch # evaluate every epoch
bf16: true # use bf16 16-bit (mixed) precision
tf32: false # use tf32 precision
learning_rate: 2.0e-4 # initial learning rate
warmup_steps: 10 # steps for a linear warmup from 0 to `learning_rate`
lr_scheduler_type: inverse_sqrt # learning rate scheduler (see transformers.SchedulerType)
# FSDP
fsdp: "full_shard auto_wrap" # add offload if not enough GPU memory
fsdp_config:
activation_checkpointing: true
# Checkpointing
save_strategy: epoch # save checkpoint every epoch
save_total_limit: 1 # limit the total amount of checkpoints
# Logging
log_level: warning # logging level (see transformers.logging)
logging_strategy: steps
logging_steps: 1 # log every N steps
report_to:
- tensorboard # report metrics to tensorboard
output_dir: /mnt/shared/Meta-Llama-3.1-8B-Instruct
Then create the training script named sft.py
that contains the fine-tuning logic:
from datasets import load_dataset
from transformers import AutoTokenizer, set_seed
from trl import (
ModelConfig,
ScriptArguments,
SFTConfig,
SFTTrainer,
TrlParser,
get_peft_config,
get_quantization_config,
get_kbit_device_map,
)
def train(script_args, training_args, model_args):
# model and tokenizer
quantization_config = get_quantization_config(model_args)
training_args.model_init_kwargs = dict(
revision=model_args.model_revision,
trust_remote_code=model_args.trust_remote_code,
attn_implementation=model_args.attn_implementation,
torch_dtype=model_args.torch_dtype,
use_cache=False if training_args.gradient_checkpointing or
training_args.fsdp_config.get("activation_checkpointing", False) else True,
device_map=get_kbit_device_map() if quantization_config is not None else None,
quantization_config=quantization_config,
)
tokenizer = AutoTokenizer.from_pretrained(model_args.model_name_or_path,
trust_remote_code=model_args.trust_remote_code,
use_fast=True)
if tokenizer.pad_token is None:
tokenizer.pad_token = tokenizer.eos_token
# training and evaluation datasets
train_dataset = load_dataset(
path=script_args.dataset_name,
name=script_args.dataset_config,
split=script_args.dataset_train_split,
)
test_dataset = None
if training_args.eval_strategy != "no":
test_dataset = load_dataset(
path=script_args.dataset_name,
name=script_args.dataset_config,
split=script_args.dataset_test_split,
)
# templatize datasets
def template_dataset(sample):
messages = [
{"role": "user", "content": sample['question']},
{"role": "assistant", "content": sample['answer']},
]
return {"text": tokenizer.apply_chat_template(messages, tokenize=False)}
train_dataset = train_dataset.map(template_dataset,
remove_columns=["question", "answer"])
if training_args.eval_strategy != "no":
test_dataset = test_dataset.map(template_dataset,
remove_columns=["question", "answer"])
# training loop
trainer = SFTTrainer(
model=model_args.model_name_or_path,
args=training_args,
train_dataset=train_dataset,
eval_dataset=test_dataset,
peft_config=get_peft_config(model_args),
processing_class=tokenizer,
)
if trainer.accelerator.is_main_process and hasattr(trainer.model, "print_trainable_parameters"):
trainer.model.print_trainable_parameters()
checkpoint = None
if training_args.resume_from_checkpoint is not None:
checkpoint = training_args.resume_from_checkpoint
trainer.train(resume_from_checkpoint=checkpoint)
trainer.save_model(training_args.output_dir)
with training_args.main_process_first(desc="Training completed"):
print(f"Training completed, model checkpoint written to {training_args.output_dir}")
parser = TrlParser((ScriptArguments, SFTConfig, ModelConfig))
script_args, training_args, model_args = parser.parse_args_and_config()
set_seed(training_args.seed)
train(script_args, training_args, model_args)
You can then create a ConfigMap from those two files so they can be mounted into the PyTorchJob pods:
oc create configmap sft --from-file=config.yaml=config.yaml --from-file=sft.py=sft.py
If you haven’t created a PersistentVolumeClaim (PVC) already as described in the “Create a workbench” section of the Fine-tune LLMs with Kubeflow Trainer on OpenShift AI article, you can create one with the following command (you may need to adjust the storageClassName
field):
cat <<EOF | kubectl apply -f -
apiVersion: v1
kind: PersistentVolumeClaim
metadata:
name: shared
spec:
accessModes:
- ReadWriteMany
resources:
requests:
storage: 500Gi
storageClassName: ocs-storagecluster-cephfs
EOF
Hereafter is the PyTorchJob from the Fine-tune LLMs with Kubeflow Trainer on OpenShift AI article that we are going to use as a baseline to compare the performance with the updated version that leverages our accelerated networking configuration:
apiVersion: kubeflow.org/v1
kind: PyTorchJob
metadata:
name: sft-baseline
spec:
nprocPerNode: "2"
pytorchReplicaSpecs:
Master:
replicas: 1
template: &template
spec:
containers:
- name: pytorch
command:
- bash
- -c
- torchrun /etc/config/sft.py --config /etc/config/config.yaml
env:
- name: HF_HOME
value: /mnt/shared/.cache
- name: HF_TOKEN
value: ""
- name: PYTORCH_CUDA_ALLOC_CONF
value: "expandable_segments:True"
- name: NCCL_DEBUG
value: INFO
image: "quay.io/modh/training:py311-cuda121-torch241"
resources:
limits: &resources
cpu: "4"
memory: 96Gi
nvidia.com/gpu: "2"
requests: *resources
volumeMounts:
- mountPath: /etc/config
name: config
- mountPath: /mnt/shared
name: shared
- name: shm
mountPath: /dev/shm
volumes:
- configMap:
name: sft
name: config
- name: shared
persistentVolumeClaim:
claimName: shared
- name: shm
emptyDir:
medium: Memory
sizeLimit: 2Gi
Worker:
replicas: 1
template: *template
It’s configured to have two pods with two GPUs attached to test intra-node and inter-node communication based on our test environment, which is detailed in the “Compare Performance” section below. You may want to adjust the topology of the job according to your own environment. Mind that you also need to set the HF_TOKEN environment variable with a valid user access token from Hugging Face.
Note
You can find the list of the supported base container images in the “training images” section of Red Hat OpenShift AI supported configurations.
Given the network configuration described in the “Configure the High-Performance Network” above, this baseline version can be updated so it uses RDMA over Ethernet (RoCE) by adding the following elements:
- The
k8s.v1.cni.cncf.io/networks
:rdmashared-net
annotation to attach the secondary network to the PyTorchJob pods. - The
NCCL_SOCKET_IFNAME
:net1
environment variable to instruct NCCL to use the network interface to communicate over this secondary network. - The
rdma/rdma_shared_device_eth
:1
in the extended resource requests and limits - Optional: the
NCCL_IB_HCA
:mlx5_1
environment variable to specify which Host Channel Adapter (HCA) NCCL should use, otherwise discovered automatically.
This corresponds to applying the following “patch” to the baseline PyTorchJob above:
apiVersion: kubeflow.org/v1
kind: PyTorchJob
metadata:
name: sft-roce
spec:
pytorchReplicaSpecs:
Master:
template: &template
metadata:
annotations:
k8s.v1.cni.cncf.io/networks: "rdmashared-net"
spec:
containers:
- name: pytorch
env:
- name: NCCL_SOCKET_IFNAME
value: "net1"
- name: NCCL_IB_HBA
value: "mlx5_1"
resources:
limits: &resources
rdma/rdma_shared_device_eth: "1"
Once the job is created, you can follow its progress by running the following command:
oc logs -l training.kubeflow.org/job-role=master -f
To validate NCCL has been properly configured, you should see the following messages being printed:
NCCL INFO NET/IB : Using [0]mlx5_1:1/RoCE [RO]; OOB net1:192.168.2.5<0>
NCCL INFO NET/IB : GPU Direct RDMA Enabled for HCA 0 'mlx5_1'
NCCL INFO GPU Direct RDMA Enabled for GPU 1 / HCA 1
NCCL INFO GPU Direct RDMA Enabled for GPU 0 / HCA 1
NCCL INFO Channel 00/0 : 2[0] -> 1[1] [receive] via NET/IB/1/GDRDMA
NCCL INFO Channel 01/0 : 2[0] -> 1[1] [receive] via NET/IB/1/GDRDMA
NCCL INFO Channel 00/0 : 0[0] -> 3[1] [send] via NET/IB/1/GDRDMA
NCCL INFO Channel 01/0 : 0[0] -> 3[1] [send] via NET/IB/1/GDRDMA
Compare performance
We’ve run the example on 2 Dell PowerEdge-R760xa nodes equipped with 2 NVIDIA A40 GPUs and 2 BlueField-3 DPUs in NIC mode each connected to a NVIDIA Spectrum-4 Ethernet switch.
The baseline PyTorch job, that uses the default OVN network completed in 5 hours, while the PyTorch job configured to use RDMA over Ethernet completed in 1 hour and 40 minutes, reducing the fine-tuning duration by a factor of 3. We’ve also run the job so it uses the secondary interface attached to the Spectrum-4 Ethernet switch, with NCCL forced to use the TCP/Socket network instead of GPUDirect RDMA, which completed in 2 hours and 30 minutes.
This is illustrated in Figures 2 and 3 with 1) the left/green curves for GPUDirect RDMA, 2) the middle/blue curves for TCP/Socket with Spectrum-4 Ethernet switch, and 3) right/red curves for default OVN network.
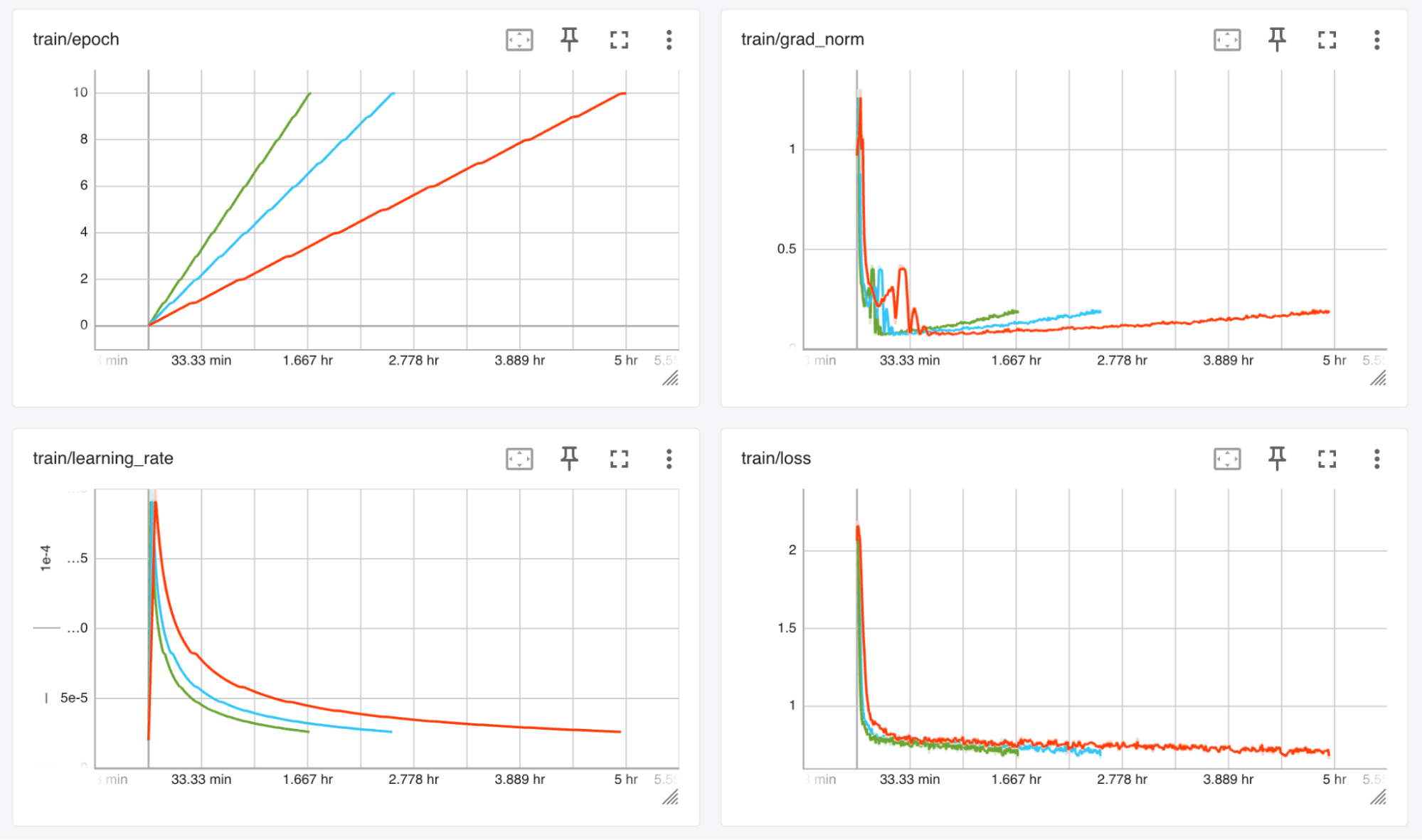
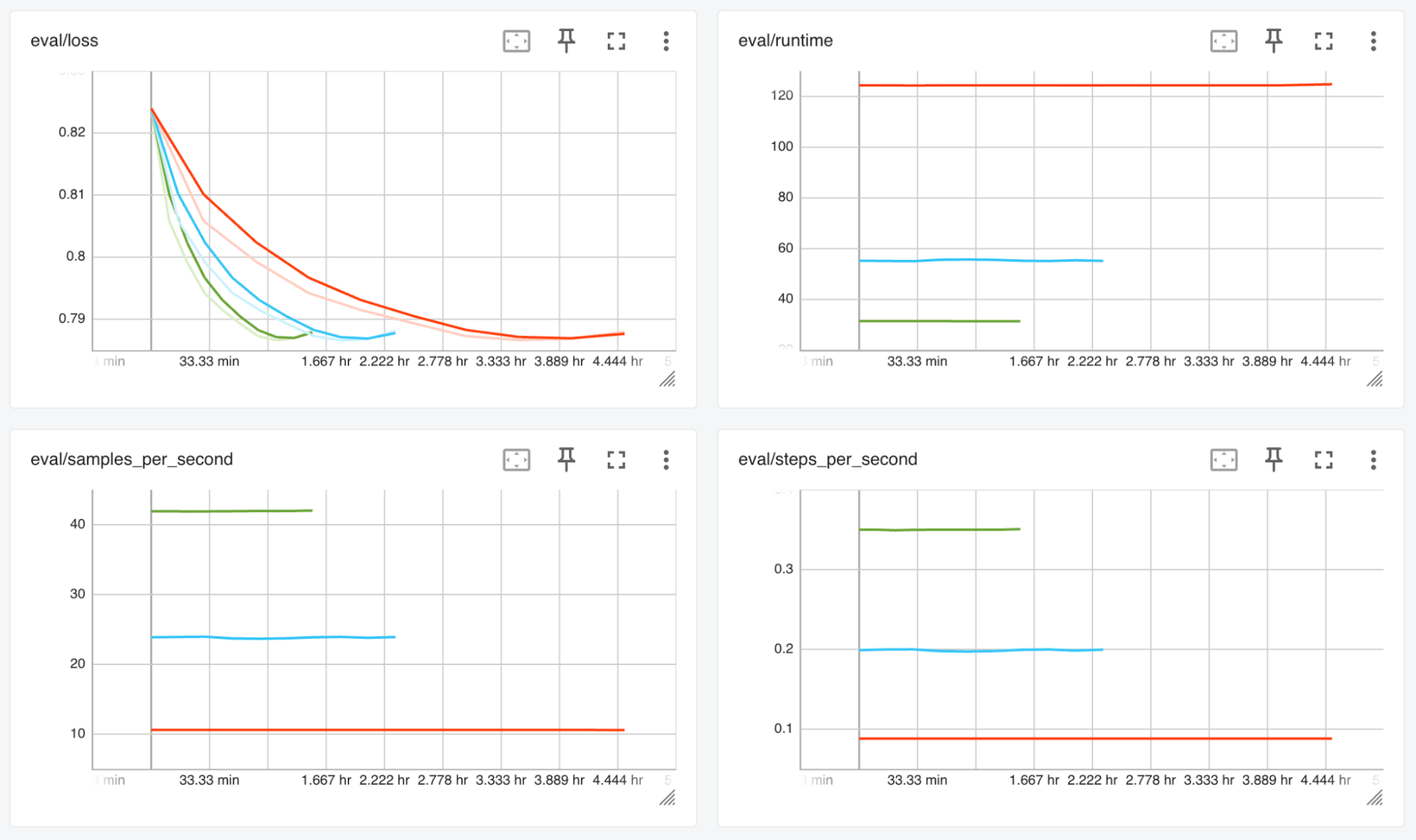
Testing with GPUDirect RDMA and different batch sizes highlights the training job is now compute-bound instead of IO-bound, as we’ve noticed in our experimentation while using the default OVN network in the Fine-Tune LLMs with Kubeflow Training on OpenShift AI article. This is illustrated in Figure 4, where the batch size varies from 32, 64, 96 and 112 while the completion time remains constant.
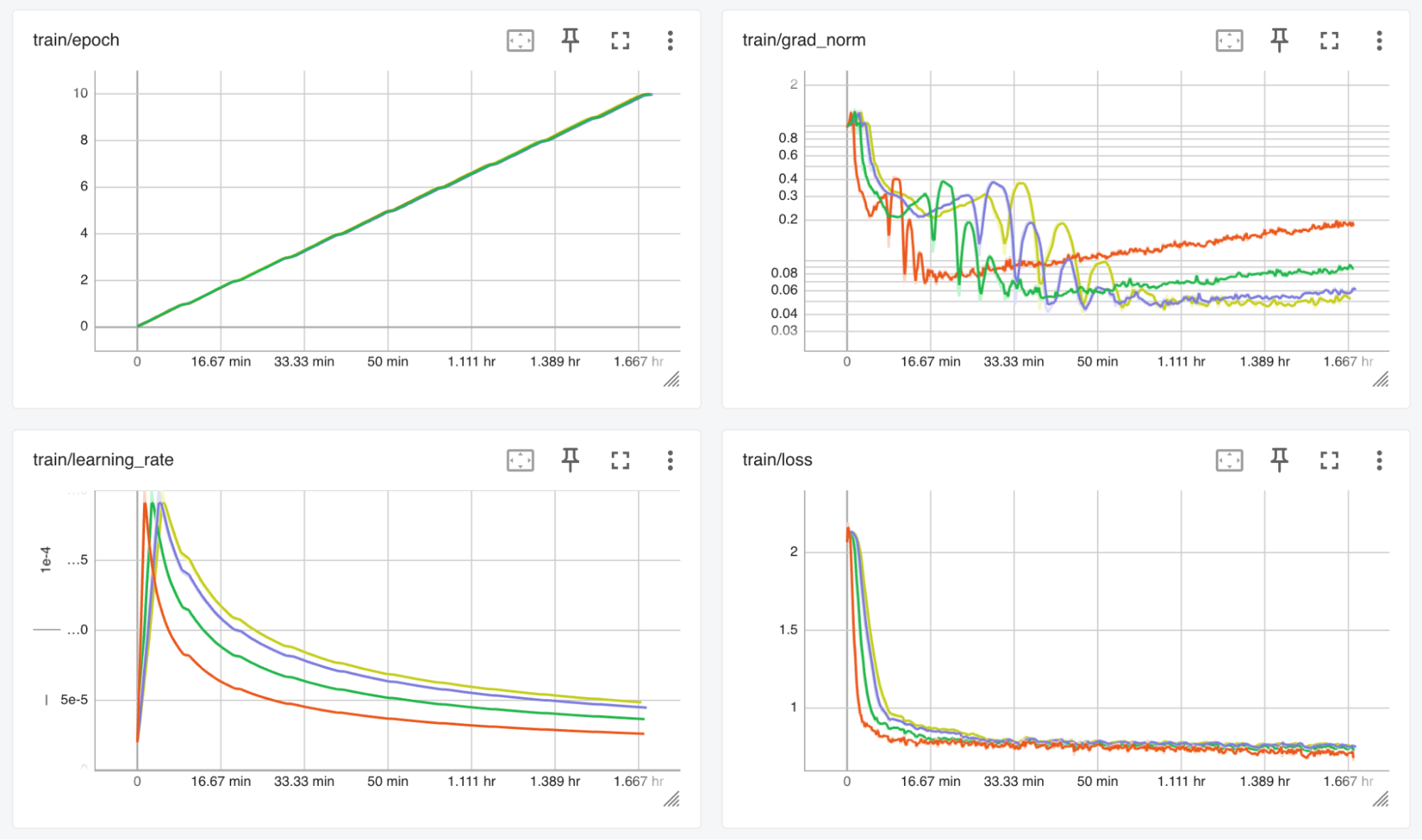
The fact the training is now compute-bound thanks to GPUDirect RDMA also enables it to benefit from the speed-up of using fused kernels from Flash Attention and Liger Kernel. This is illustrated in Figure 5 with 1) left/green curves Flash Attention + Liger Kernel, 2) middle/blue curves Flash Attention only and 3) default attention implementation, no optimized kernels.
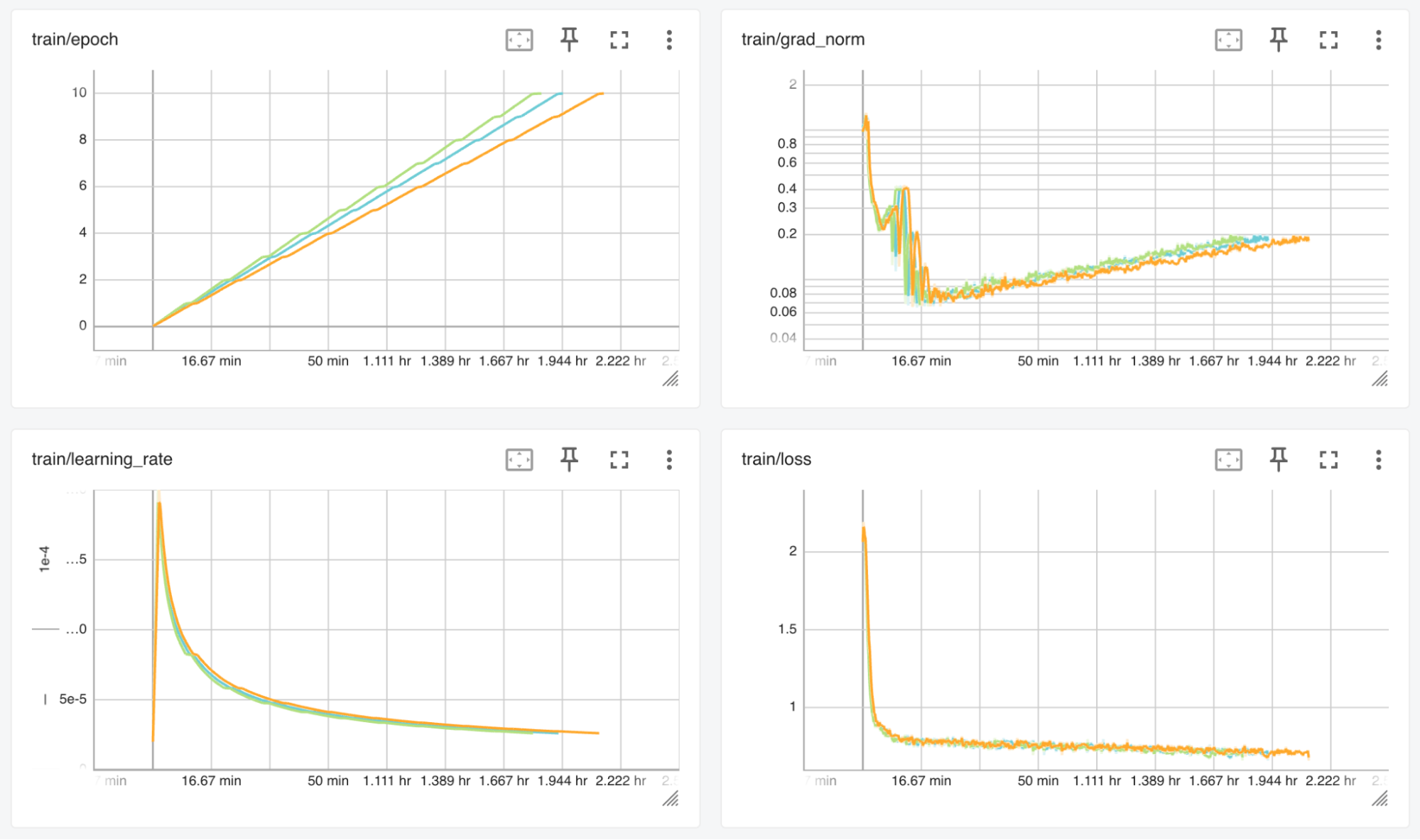
Conclusion
Training LLMs — and deep learning models in general — is a very iterative process by nature. Being able to speed up the development cycle during the experimentation phase is a competitive advantage, and removing communication bottlenecks is essential to take the most of the massive compute power of GPUs.
Using a typical LLM fine-tuning example, we’ve demonstrated how NVIDIA GPUDirect RDMA can remove those communication bottlenecks for distributed training. We've also highlighted how important it is to invest into a networking platform that matches the compute power of GPUs.
NVIDIA GPUDirect RDMA enables the acceleration of other use cases like distributed model serving. NVIDIA GPUDirect serves as the foundation for other technologies like GPUDirect Storage, which accelerates how data moves between storage and GPU memory.
Thanks to the long-term partnership between NVIDIA and Red Hat, and with the many communities powering a global open source software ecosystem, OpenShift AI can deliver a versatile yet powerful AI/ML platform that supports the best of hardware and software, with high-performance NVIDIA networking platforms such as Spectrum-X and the leading container orchestration platform OpenShift.
To learn more about OpenShift AI, visit red.ht/openshift_ai.
Check out the AI on OpenShift site for reusable patterns and recipes: https://ai-on-openshift.io/.