The rapid advancement of large language models (LLMs) has revolutionized how we interact with and utilize artificial intelligence. From generating creative content to answering complex questions, LLMs have demonstrated remarkable capabilities. However, training LLMs requires vast computational resources and massive datasets, posing significant financial and logistical challenges. Furthermore, aligning these models with ethical values and confirming that their outputs are safe and unbiased remains a complex and ongoing problem.
DeepSeek, a Chinese artificial intelligence company, launched its DeepSeek-R1 open source LLM in January 2025 and revolutionized the AI community with a fast, high-performance, and low-cost model. But how was DeepSeek-R1 created?
Creating a large language model
Let's look at how LLMs are generally created. The LLM creation process involves several phases.
Phase 1: Data collection and processing
Data collection is the crucial first step, focusing on gathering a massive and diverse dataset of text relevant to the intended capabilities of the LLM. This involves identifying reliable sources like books, websites, articles, code repositories, and potentially specialized datasets depending on the LLM's purpose. The emphasis is on both quantity and quality. A larger dataset generally leads to better performance, but the data must also be representative, unbiased, and as free of errors as possible. This phase often involves web scraping, utilizing APIs, and collaborating with data providers to acquire the necessary text data.
Data processing follows data collection, preparing the raw text for model training. This is a multi-stage process:
- Begins with cleaning, where irrelevant information, duplicates, and errors are removed, and missing data is handled.
- Normalization then standardizes the text, often involving lowercasing, punctuation removal, and handling special characters.
- Finally, tokenization breaks the text into smaller units, like words or sub-word units, that the model can understand. This stage is critical as it directly impacts how the LLM interprets and learns from the data, and efficient tokenization is essential for managing the computational demands of training.
Phase 2: Model selection
This phase involves choosing the appropriate architecture and framework for the LLM. The transformer architecture has become dominant due to its ability to effectively handle long-range dependencies in text and parallelize computations, making it ideal for processing the massive datasets required for LLMs. Within the transformer framework, decisions must be made regarding the specific configuration, including the number of layers, attention heads, and embedding dimensions. These choices directly impact the model's capacity and performance, often requiring experimentation and careful consideration of available computational resources.
Phase 3: Model training
Model training is the computational heart of LLM development, where the chosen model architecture is exposed to the prepared training data and learns to predict the next word in a sequence. This process involves feeding the tokenized data into the model in batches and iteratively adjusting the model's parameters (weights) to minimize the difference between its predictions and the actual next words. Training LLMs requires immense computational power, often utilizing clusters of high-performance GPUs and specialized software frameworks, and a lot of money.
The training process is highly resource-intensive and time-consuming, often taking weeks or even months to complete for the largest models. Key factors influencing training include the size of the dataset, the model's complexity, and the computational resources available.
Phase 4: Reinforcement learning from human feedback
Reinforcement learning from human feedback, RLHF is a machine learning technique that trains a model to align with human preferences. It involves training a reward model to represent these preferences, which can then be used to train other models through reinforcement learning. This reward is based on human feedback, which can be given in the form of ratings, rankings, or other types of input. The model learns to perform the task by trying to maximize the reward it receives.
Figure 1 illustrates the steps for implementing this process.
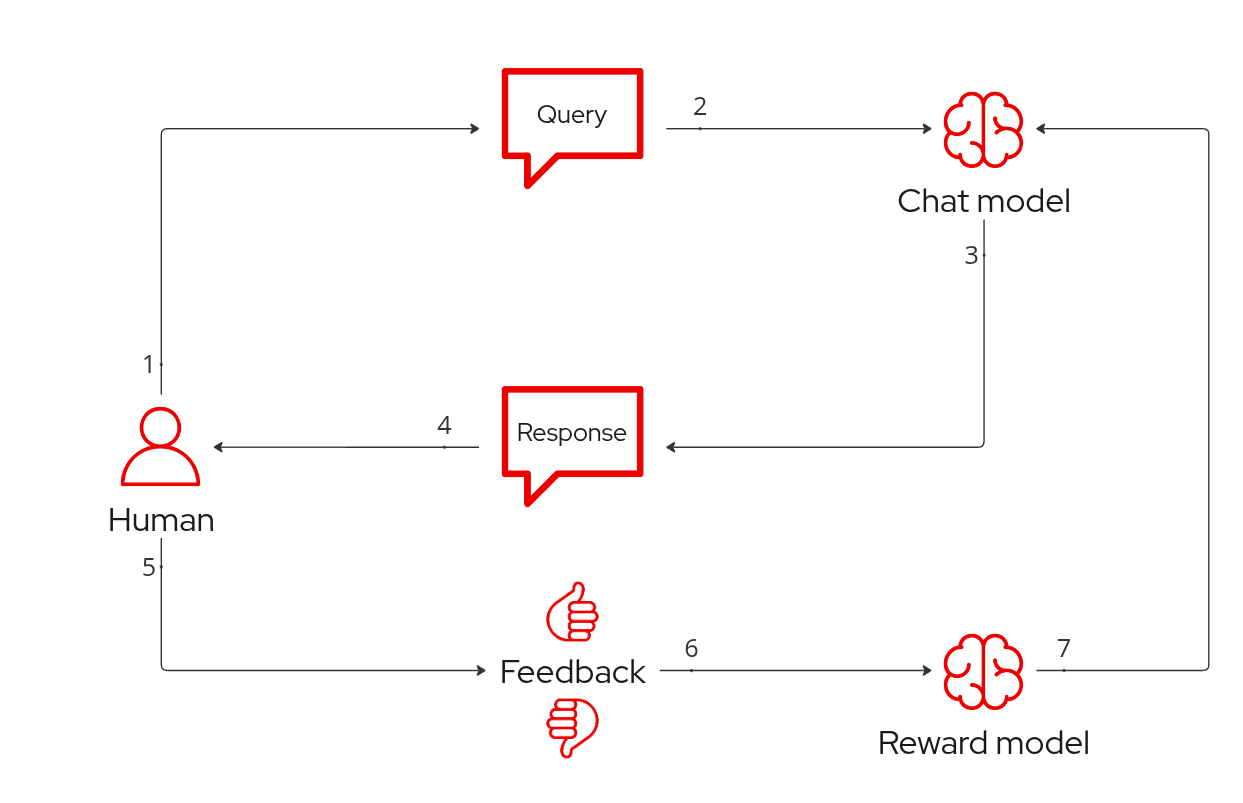
In greater detail, the steps are as follows:
- The human provides a query or prompt to the chat model.
- The chat model receives and processes the human's query.
- A response to the query is generated by the LLM.
- The LLM's output is presented as the response of the input query.
- The human provides feedback on the quality of the response.
- The feedback is used to train a reward model.
- The reward model guides further the chat model training via reinforcement learning.
RLHF is recognized as the industry standard technique for creating LLMs that are most likely to produce content that is truthful, harmless, and helpful. It's a powerful tool for improving AI. But it's not a perfect solution, and it requires careful implementation and consideration of ethical implications.
How DeepSeek-R1 was created
According to DeepSeek's published paper, unlike the traditional model building process, the company created its R1 model exclusively using reinforcement learning algorithms for complex reasoning tasks without any supervised model training and/or fine-tuning beforehand.
Reinforcement learning (RL) is a machine learning technique where an agent learns to make decisions in an environment by maximizing rewards. It's one of the core approaches in machine learning, including supervised learning and unsupervised learning, and draws from the optimal control theory. Figure 2 shows the Markov decision process for reinforcement learning.
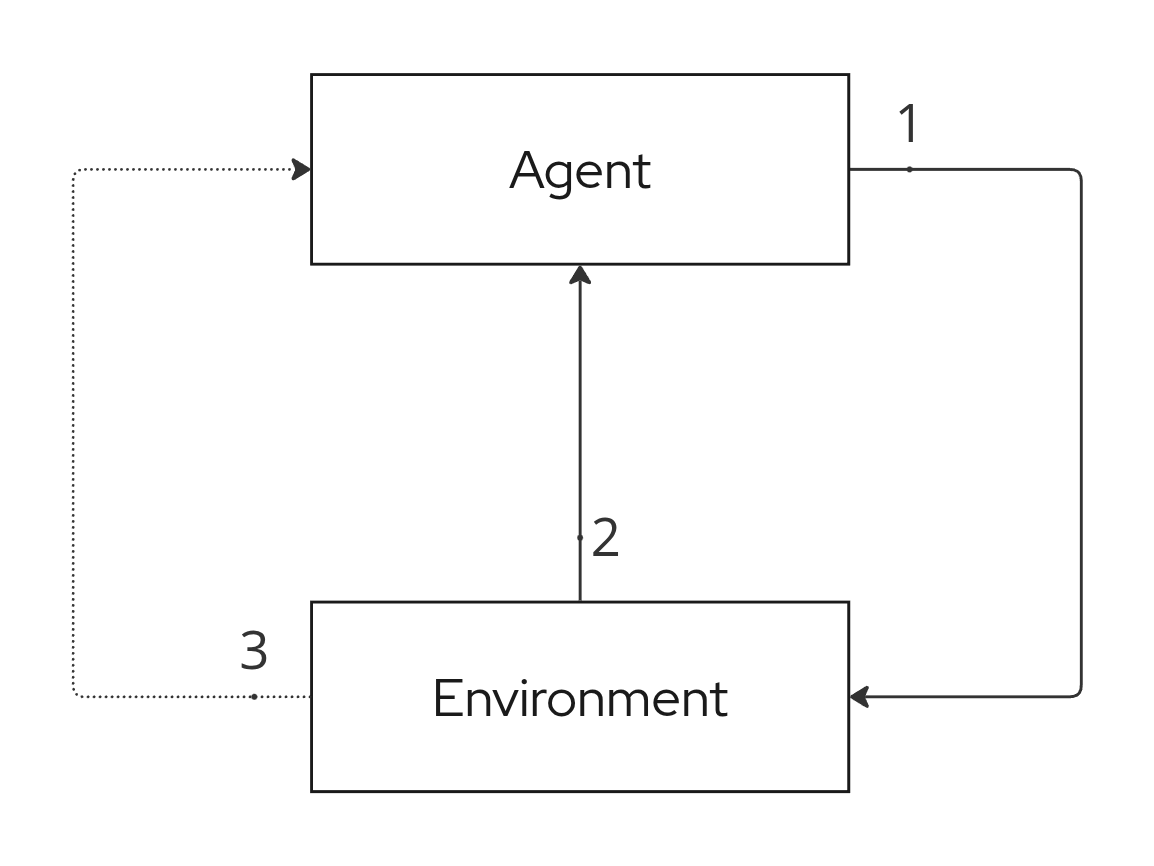
The steps can be described as follows:
- The reinforcement learning agent learns about a problem by interacting with its environment.
- The environment provides information on its current state. The agent then uses that information to determine which actions(s) to take.
- If that action obtains a reward signal from the surrounding environment, the agent is encouraged to take that action again when in a similar future state.
To save the training costs of RL, DeepSeek researchers adopted the Group Relative Policy Optimization (GRPO) algorithm. Figure 3 illustrates this algorithm, from a general perspective.
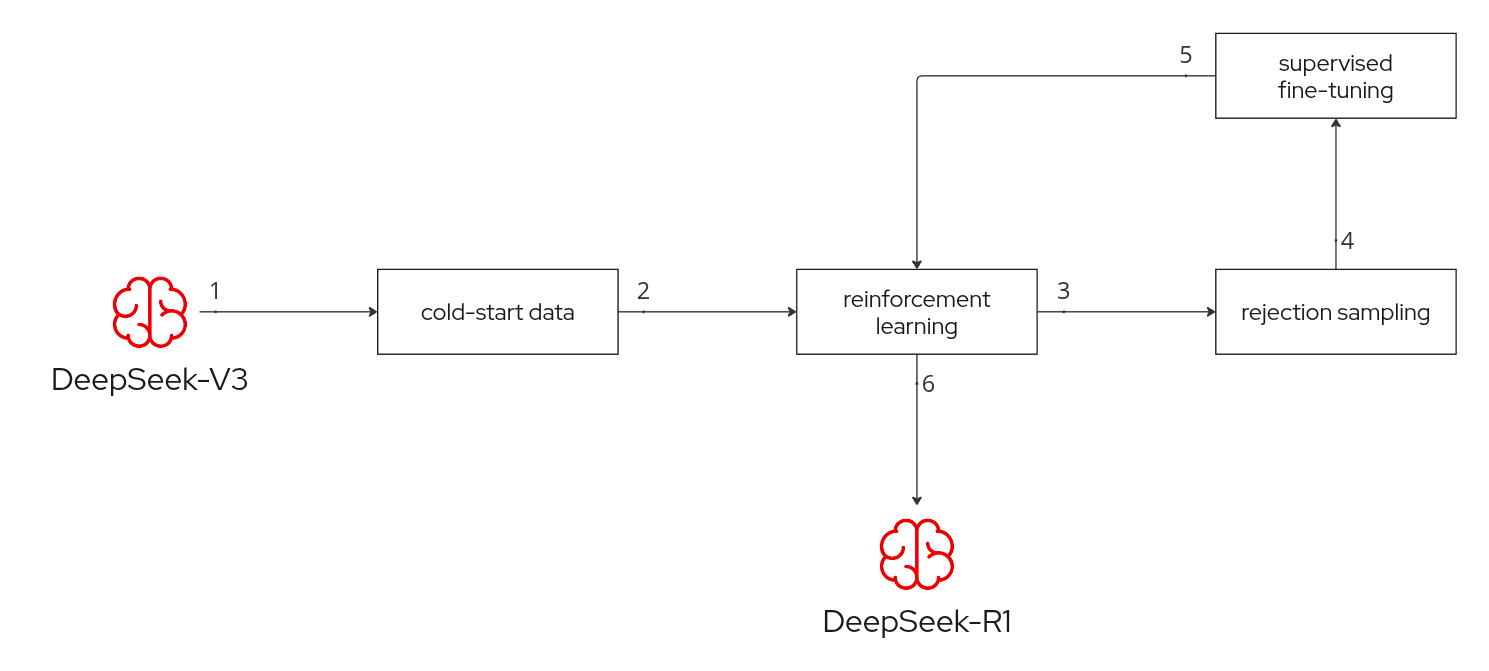
As the diagram indicates, the phases of this algorithm are as follows:
- The DeepSeek-V3-Base was used as the base model.
- The DeepSeek-V3 base model was fine-tuned with thousands of cold-start data points to establish a solid foundation. Note that thousands of cold-start data points are a tiny fraction compared to the millions or even billions of labeled data points typically required for supervised learning at scale.
- Reinforcement learning was applied with a focus on enhancing the model’s reasoning capabilities, particularly in reasoning-intensive tasks such as coding, mathematics, science, and logic reasoning, which involve well-defined problems with clear solutions.
- When reasoning-driven RL converges, the rejection sampling technique was used to select the best examples from the last successful RL run.
- The newly generated data is now merged with the supervised data. Unlike the initial cold-start data, which primarily focuses on reasoning, this stage incorporates data from other domains to enhance the model’s capabilities in writing, role-playing, and other general-purpose tasks.
- The model is then fine-tuned with the new data and undergoes a final RL process across multiple prompts and controlled scenarios.
- After two epochs, DeepSeek-R1 was created.
To further align the model with human preferences and answer questions in a similar way to an assistant, a secondary reinforcement learning stage was implemented to improve the usefulness and harmlessness of the model, while refining its reasoning capabilities.
Figure 4 shows a simple example of a question, answer, and its rewards based on whether the answer is correct and whether the formatting of the answer is correct.
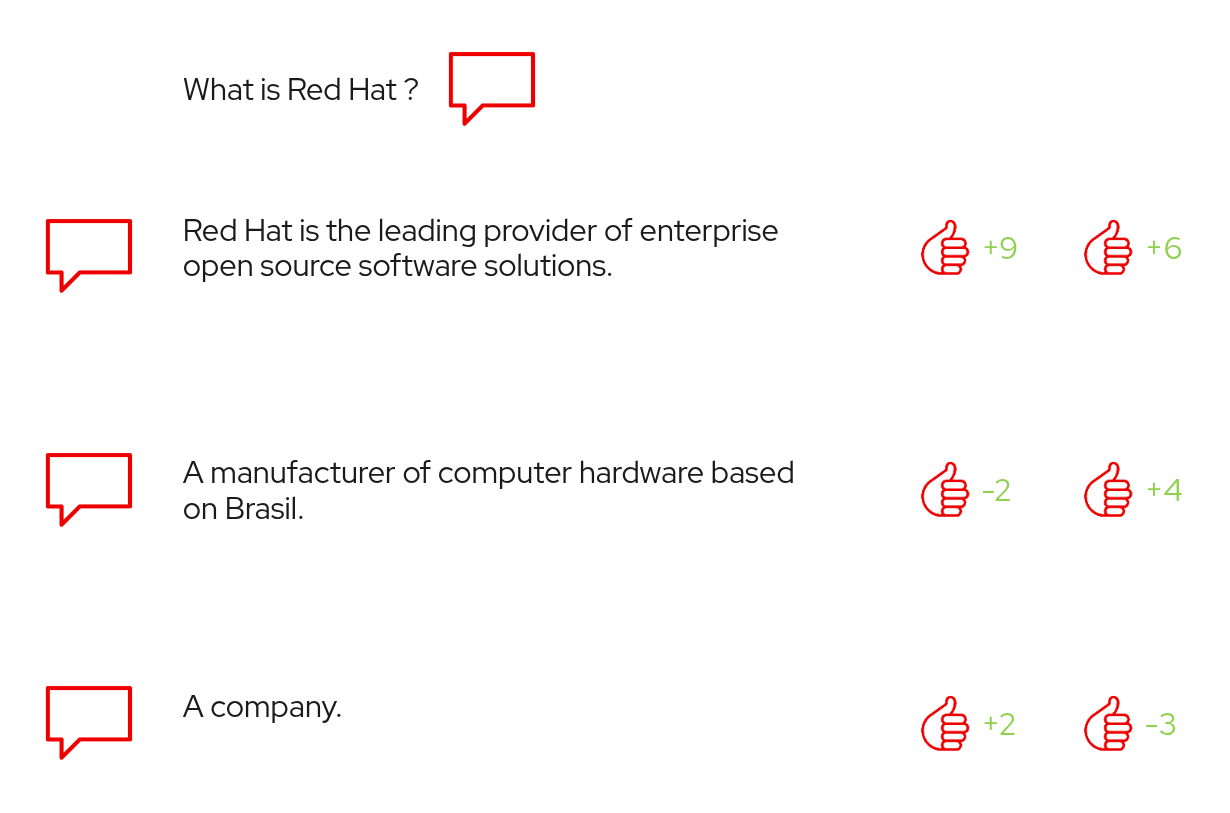
Another important aspect of the training was the use of chain of thoughts (CoT). This is a technique that mirrors human reasoning, facilitating the systematic resolution of problems through a coherent series of logical deductions.
Without the reward model in the process, the final model had to learn to arrive at a solution to a problem on its own. One of the ways the model learned to receive more rewards was to think more about the problem. More reasoning brought better results.
Taken from the article, Figure 5 is an example of what the model calls an "aha moment," when it realizes that its current thinking is flawed. So it backtracks and starts over.

Get started with DeepSeek
Red Hat OpenShift AI provides a robust and scalable platform that significantly simplifies the deployment and management of complex artificial intelligence applications, including LLMs like DeepSeek-R1. Its containerization and orchestration capabilities allow users to package DeepSeek and its dependencies into portable containers, ensuring consistent performance across various environments.
The development of the DeepSeek-R1 represents a significant leap forward in accessible and high-performance AI. By effectively leveraging RL during the training process, the researchers demonstrated a pathway to creating models that not only rival but surpass established LLMs, such as OpenAI's o1 and Meta's Llama across a multitude of benchmarks.
This advancement democratizes access to state-of-the-art language processing, empowering researchers, developers, and enthusiasts to innovate without the prohibitive costs and constraints often associated with proprietary models. The success of DeepSeek-R1 highlights the potential of optimized training techniques to unlock new levels of efficiency and performance in open source LLMs.