Imagine having the ability to customize a Large Language Model (LLM) to talk like your company, know about your business, and help you fix your specific business challenges with precision. This is not something from the future. It’s the current reality of fine-tuning LLMs at scale, a capability that is transforming how organizations use AI to get ahead of their competitors.
In this series, we'll share our latest findings on fine-tuning LLMs with Red Hat OpenShift AI (RHOAI). These insights will be valuable whether you're customizing models for specific use cases or scaling AI operations across multiple cloud environments. In this article, we will introduce our model fine-tuning stack and discuss how we run performance and scale validation of the fine-tuning process.
Using OpenShift AI to build applications
Red Hat OpenShift AI provides enterprises a comprehensive platform for building and deploying impactful AI applications. It is a central management system for coordinating everything from data ingestion to model serving across hybrid cloud environments, allowing organizations to focus on creating value rather than managing infrastructure.
Our team, Performance and Scalability for AI Platforms (PSAP), continues to advance LLM fine-tuning capabilities among other innovations. We're developing solutions that enable enterprises to leverage AI effectively while maintaining robust security and compliance standards essential for business operations.
AI infrastructure setup has traditionally presented significant challenges. Many ML engineers have encountered difficulties with dependencies and resource orchestration. RHOAI addresses these pain points directly, enabling users to fine-tune models and deploy applications with greater efficiency.
This approach transforms AI development into a reliable, enterprise-grade workflow that operates seamlessly across hybrid cloud environments. It eliminates the need to choose between innovation and stability or between speed and security.
Model fine-tuning stack
Imagine a musician carefully adjusting their instrument until it produces the perfect note, or an F1 engineer tweaking the engine for the best performance.
In the AI domain, we adapt powerful, pre-trained models for specialized tasks. It's comparable to providing a talented generalist with expertise in your specific business domain.
The challenge lies in the resources required to train these large models from scratch. The computational and financial demands are substantial, making this approach not feasible for most organizations. Fine-tuning has, therefore, become the preferred method in modern AI development. This impact has been so great that we now refer to initial model training as "pre-training" with fine-tuning representing the important final stage.
OpenShift AI streamlines this process, supporting both custom images and public repository integration. We've incorporated fms-hf-tuning, a robust toolkit developed by our colleagues at IBM Research, which leverages HuggingFace SFTTrainer and PyTorch FSDP. This versatile solution can be utilized as a Python package or implemented in our experiments as a container image with enhanced scripting capabilities.
Technical note: Our experiments use different fms-hf-tuning images based on the RHOAI version (more on that later).
We're going to take a deep dive into exploring three different flavors of fine-tuning on OpenShift AI:
- Full parameter fine-tuning: The conventional method that adjusts all parameters within the network.
- LoRA (low rank adapters): An efficient approach that achieves fine-tuning with significantly reduced resource requirements.
- QLoRA (quantized low rank adapters): One of the latest advancements in resource-efficient fine-tuning techniques.
Each methodology offers specific advantages and limitations. We'll examine when and why you might select one approach over another based on your particular use case.
Full parameter fine-tuning
Think of full parameter fine-tuning as providing your LLM with a comprehensive update rather than a minor adjustment. Unlike the more efficient approaches, LoRA and QLoRA (which we'll discuss later), this method adjusts every parameter in your neural network (see Figure 1).
What makes this different from building a model from scratch? In this approach, model weights aren't randomly initialized. Instead, these weights already encode valuable features. With full parameter fine-tuning, you're working with a model that already has a solid foundation. You're simply adapting it to your specific requirements.
What makes full parameter fine-tuning distinctive?
- Precision through complete control: By adjusting every parameter, you're effectively teaching the model to understand your domain thoroughly. Your task-specific dataset becomes the model's comprehensive training program.
- Resource-intensive but valuable: This process requires significant computational resources and time. However, for applications where accuracy is essential, this investment often provides the best returns.
- Enhanced task-specific performance: Upon completion, your model demonstrates exceptional capability in its specialized task. It effectively transforms a general-purpose AI into one with expertise in your specific domain.
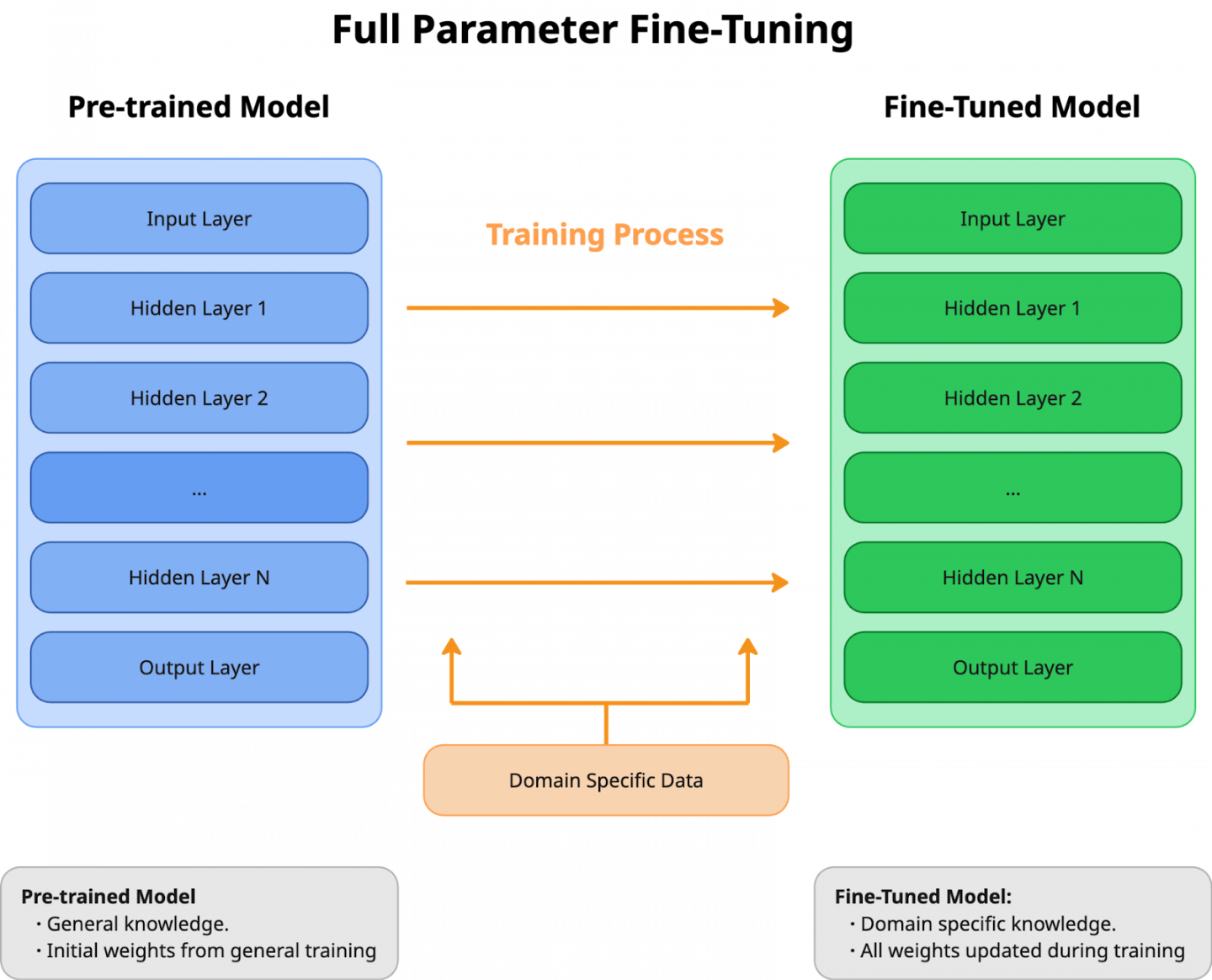
Low-rank adaptation, LoRA
Low-rank adaptation (LoRA) is an efficient approach within the parameter efficient fine-tuning (PEFT) family of algorithms. While full parameter fine-tuning modifies the entire model, LoRA adopts a more targeted strategy (see Figure 2).
The innovative aspect of LoRA is that it preserves the original model parameters and introduces two compact matrices (e.g., adapters) that work together to create the necessary adjustments. The original model remains unchanged while still providing fine-tuning benefits, offering efficiency without sacrificing effectiveness.
LoRA's effectiveness centers on two key parameters:
- rank dimension (r): This represents the complexity of your adjustments. A smaller r-value requires less memory but may offer less precise control.
- alpha (scaling factor): This determines the intensity of your adjustments on the final output.
Advantages of the LoRA algorithm:
- More efficiency: By focusing on a subset of parameters, LoRA completes training significantly faster than full parameter methods.
- Resource optimization: LoRA's streamlined approach reduces GPU memory requirements, enabling fine-tuning of models that would otherwise be too large to process.
- Versatility: LoRA allows you to develop different versions of your model for various tasks by simply exchanging adapters, providing flexibility and efficiency.
- Strategic focus: LoRA typically concentrates its modifications in the attention layers, where much of the model's critical processing occurs. This targeted approach maximizes impact while minimizing resource usage.
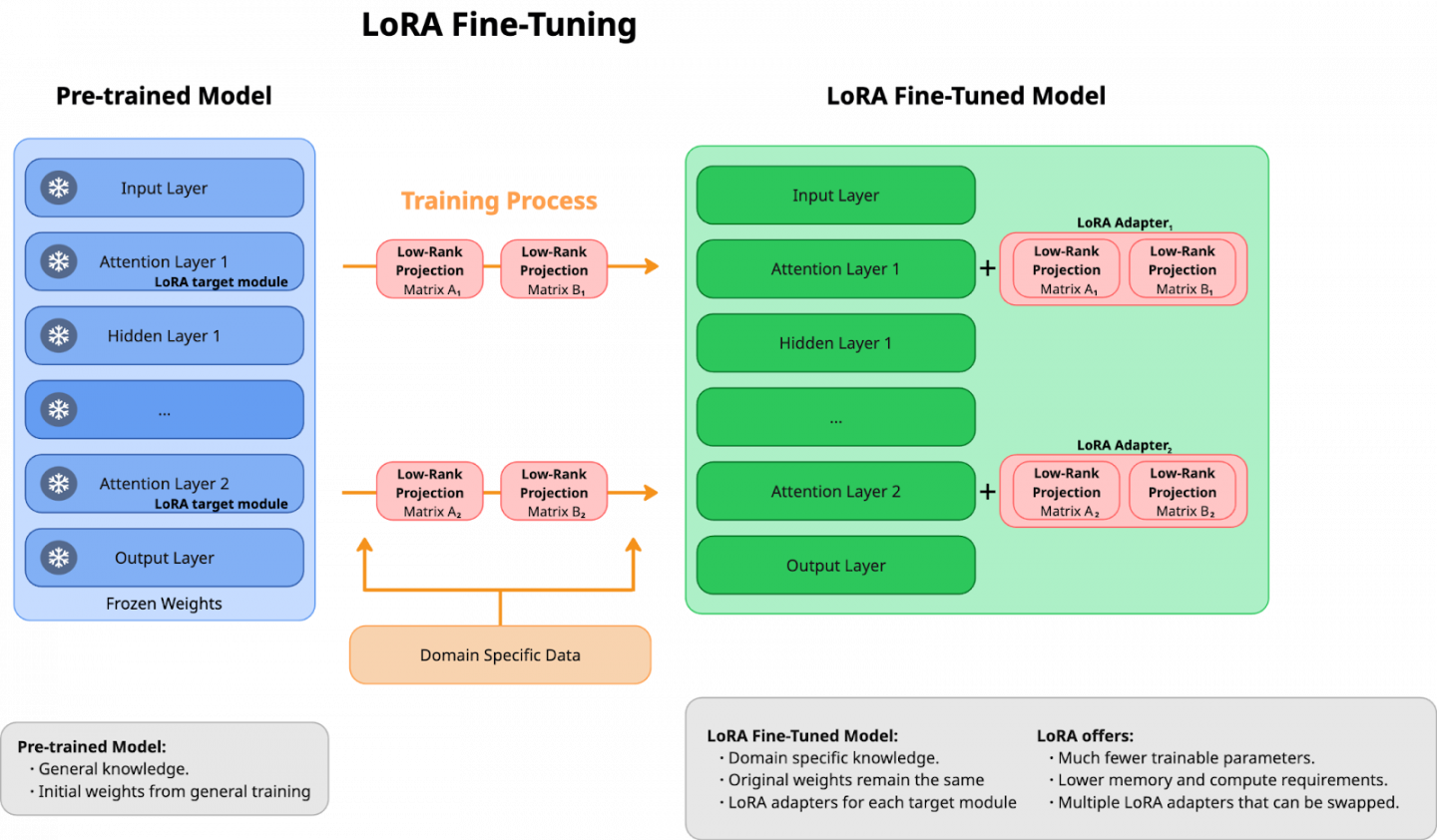
Quantized low-rank adaptation, QLoRA
Quantized low-rank adaptation (QLoRA) is a highly efficient approach to fine-tuning. The key innovation is quantization, storing model weights in 4-bit precision rather than standard full precision (see Figure 3).
However, while the model weights are compressed, QLoRA performs its learning operations in full precision. During each training update, the model temporarily returns to full precision, makes necessary adjustments, and then compresses again, effectively balancing efficiency with accuracy.
Key advantages of QLoRA, similar to LoRA:
- Memory efficiency: Substantially reduces GPU memory requirements by keeping the parameters in their quantized form most of the time.
- Accessibility: Enables fine-tuning of substantial models on consumer-grade GPUs, making advanced AI development more accessible.
- Strategic precision: Maintains full precision for critical low-rank matrices where accuracy is most important.
Processing time is the primary trade-off. The quantization and dequantization processes add additional steps to the training. However, for many applications, this is a reasonable compromise for the ability to work with models that would otherwise require specialized hardware.
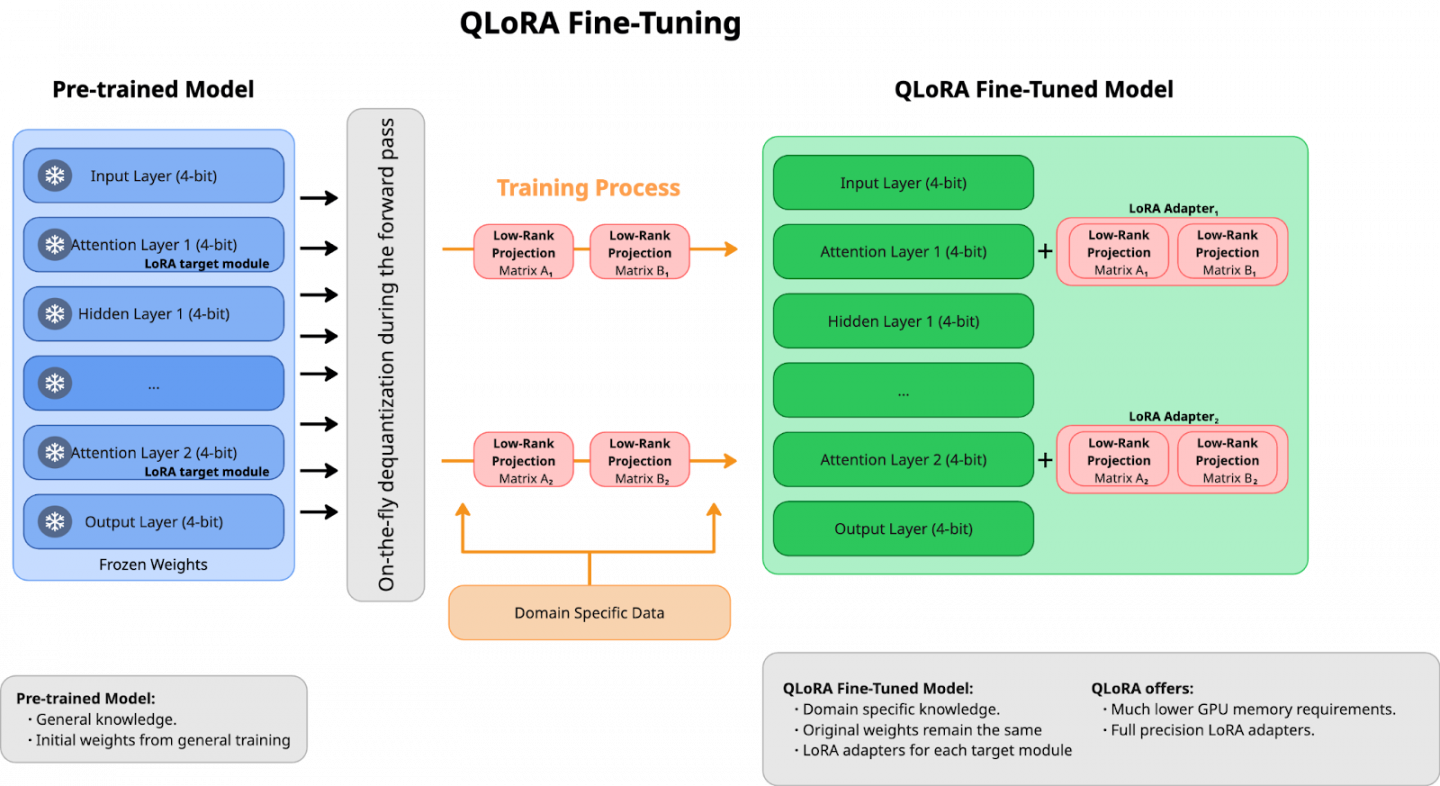
These tests ran on a Red Hat OpenShift cluster with 4xH100-80GB NVIDIA GPUs. To run these tests in an automated, transparent, and reproducible manner, we use test orchestrator for performance and scalability of AI platforms (TOPSAIL), which uses a combination of Python code for orchestration and Red Hat Ansible Automation Platform roles for cluster control.
Training results
Diving deeper into our journey, we employed the fms-hf-tuning image to conduct our fine-tuning experiments. We put three distinct approaches through their paces: full fine-tuning, LoRA, and QLoRA. Let's pull back the curtain on our test settings and unpack what we discovered.
Technical note: We focused specifically on benchmarking the fine-tuning infrastructure rather than evaluating the model’s accuracy through LLM testing. The main objective was to assess the performance of RHOAI fine-tuning stack and compare it against internal benchmarks. Think of it as testing the kitchen rather than rating the meal.
For this exploration, we examined results across three RHOAI versions:
- RHOAI 2.16 paired with fms-hf-tuning v2.2.1
- RHOAI 2.17 paired with fms-hf-tuning v2.5.0
- RHOAI 2.18 paired with fms-hf-tuning v2.6.0
In upcoming articles, we'll dive into the technical implications of these findings. But for now, we're keeping our spotlight on the automation framework. While we gathered a different kind of metrics during our fine-tuning tests, we chose three that we considered the most informative in terms of performance:
Train runtime: How long the process takes.
Train throughput: How efficiently it processes data.
Maximum GPU memory usage across all GPUs: How resource-hungry the process becomes.
Let's dive into what these numbers tell us.
The first testing setup
When your AI needs a complete wardrobe change, not just a new tie. In the first test scenario, the selected method is full parameter fine-tuning, which is the traditional approach where all model parameters are updated during training. While this method typically achieves the best inference results, it requires significant computational resources since the entire neural network is modified.
This test consists of running full parameter fine-tuning for a given set of models in an OpenShift cluster. The test settings are as follows:
- Dataset: Cleaned Alpaca Dataset
- Replication factor of the dataset (where 1.0 represents the full dataset): 0.2
- Number of accelerators: 4xH100-80GB NVIDIA GPU
- Maximum sequence length: 512
- Epochs: 1
The list of fine-tuned models:
- ibm-granite/granite-3b-code-instruct
- ibm-granite/granite-8b-code-base
- instructlab/granite-7b-lab
- meta-llama/Llama-2-13b-hf
- meta-llama/Meta-Llama-3.1-70B
- meta-llama/Meta-Llama-3.1-8B
- mistralai/Mistral-7B-v0.3
- mistralai/Mixtral-8x7B-Instruct-v0.1
Our earlier full parameter fine-tuning tests revealed intriguing regressions (which we'll dissect in future articles). But for now, let's focus on our comparative analysis across the three RHOAI versions. As mentioned, each version comes paired with its own fms-hf-tuning image, and Topsail proved to be our secret weapon, allowing us to automate fine-tuning evaluations while flexibly adjusting GPU counts and hyperparameters to fit various scenarios.
The first thing that one can notice in these initial results is that not all of the models manage to finish the training loop, as shown in Figure 4. The reason behind this is that for full fine-tuning, all of the weights are loaded into memory at full precision and actively participate in both forward and backward passes, receiving gradient updates after each step, triggering OOM errors for very large models (i.e., hitting the hard ceiling of your GPU's VRAM capacity).
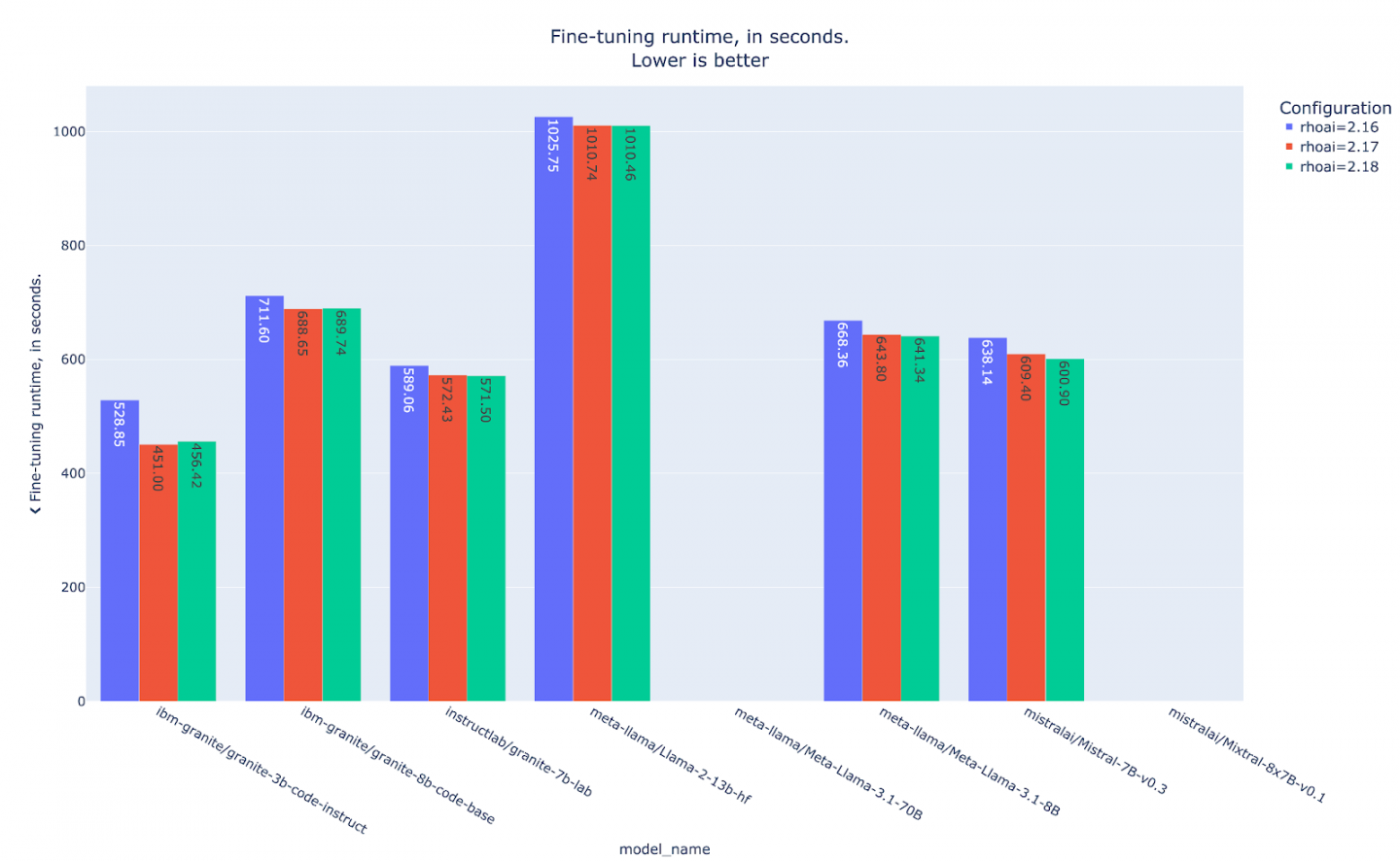
Another interesting takeaway for these initial results is that the training runtime seems to have decreased in the latest RHOAI iterations compared to the baseline RHOAI 2.16 (paired with fms-hf-tuning v2.2.1).
Examining the graph in Figure 5, which shows GPU memory utilization patterns across the different RHOAI versions, reveals a consistent footprint despite the reduced training runtime. This interesting contradiction suggests that while computational efficiency has improved, memory allocation strategies remain largely unchanged.
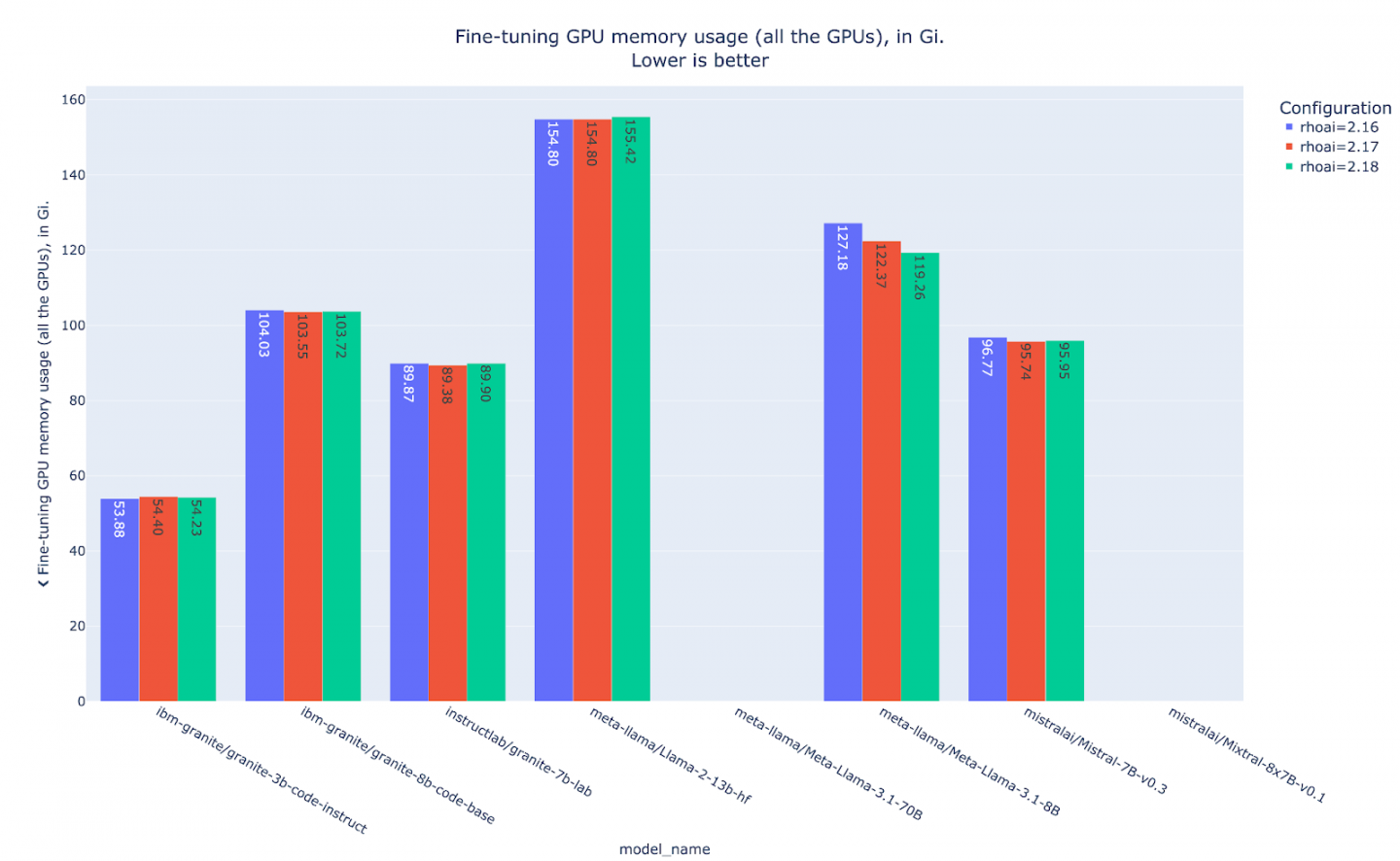
Analysis of the throughput shown in Figure 6 reinforces our earlier observations: the latest RHOAI iterations demonstrate higher throughput compared to the baseline RHOAI 2.16. This consistent pattern of improved data processing efficiency aligns with the reduced runtime findings, confirming that the performance enhancements are systematic rather than anomalous.
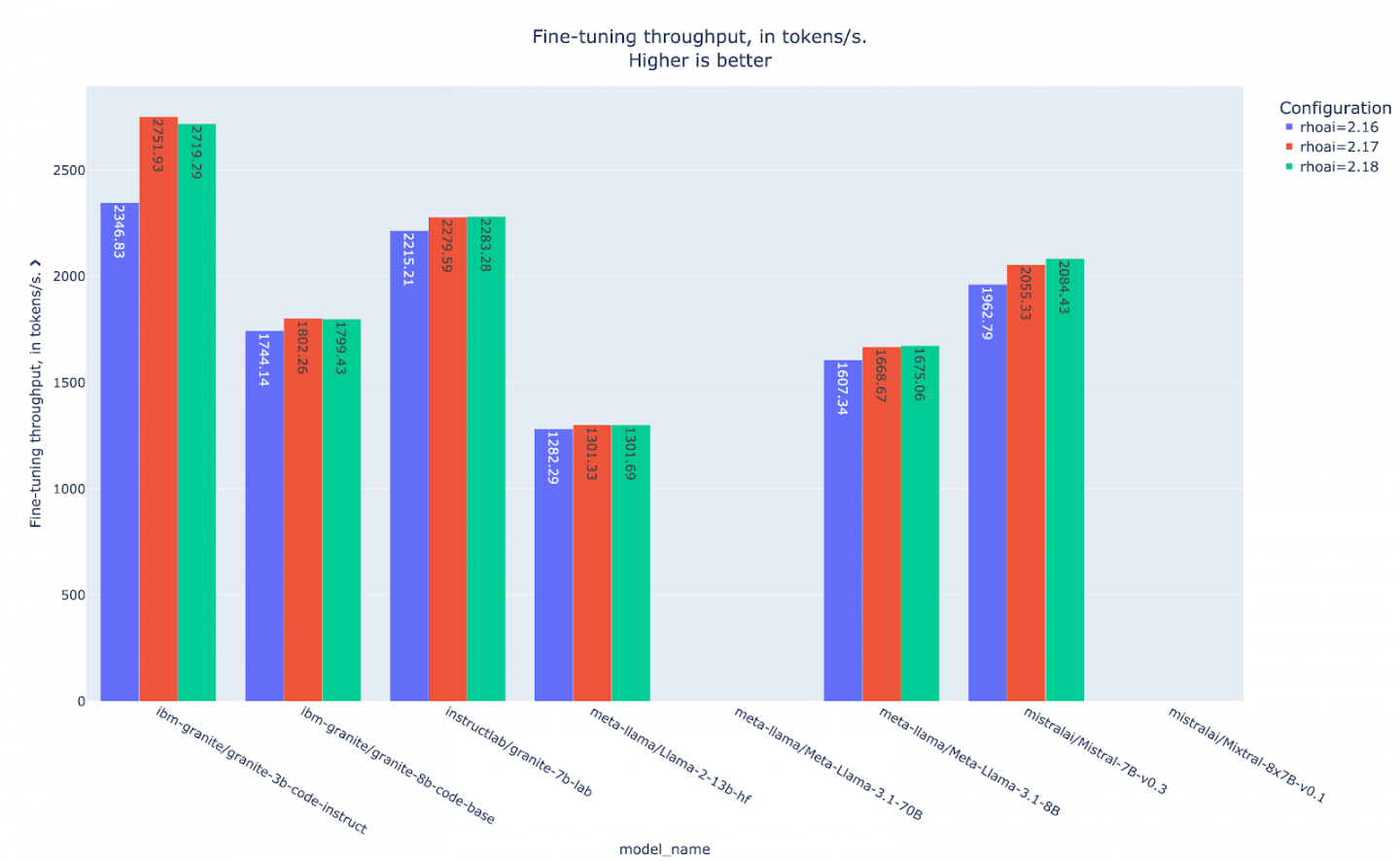
The second testing setup
LoRA's fine-tuning magic has a big impact but small footprint. The second testing setup uses low-rank adaptation (LoRA), which is a parameter-efficient fine-tuning algorithm that works by updating only an additional small subset of the model's parameters rather than modifying all of them.
This test consists of running LoRA fine-tuning for a given set of models in an OpenShift cluster. The LoRA algorithm uses a rank (r) of 4 and a scaling factor (alpha) of 16. The test settings are the following:
- Dataset: Cleaned Alpaca Dataset
- Replication factor of the dataset (where 1.0 represents full dataset): 0.2
- Number of accelerators: 4xH100-80GB NVIDIA GPU
- Maximum sequence length: 512
- Epochs: 1
The list of fine tuned models:
- ibm-granite/granite-3b-code-instruct
- ibm-granite/granite-8b-code-base
- instructlab/granite-7b-lab
- meta-llama/Llama-2-13b-hf
- meta-llama/Meta-Llama-3.1-70B
- meta-llama/Meta-Llama-3.1-8B
- mistralai/Mistral-7B-v0.3
- mistralai/Mixtral-8x7B-Instruct-v0.1
LoRA unlocks a critical performance advantage, substantially larger batch sizes that drive higher throughput rates. This creates an interesting performance dichotomy worth mentioning. In compute-bounded environments (i.e., where you have sufficient VRAM but limited computational power) LoRA can actually underperform compared to full fine-tuning due to the computational overhead in its forward pass implementation. However, the paradigms flip in memory-bounded scenarios (i.e. where VRAM capacity becomes the bottleneck).
LoRA is able to demonstrate superior performance by reducing the parameter footprint in in three key areas: minimizes trainable parameters, shrinks optimizer state storage requirements, and reduces gradient accumulation memory needs.
This fundamental trade-off highlights the importance of correctly diagnosing your infrastructure’s bottlenecks before selecting a fine-tuning approach since the optimal technique depends entirely on whether your system hits computational or memory limits first.
With LoRA properly introduced, we can jump right into examining the results. First we'll tackle the training runtime results shown in Figure 7. Now, the two largest models that hit OOM in the full fine-tuning scenario can complete the training. Note that the LoRA results are slower compared to the previous full fine-tuning experiment, which is expected in our cluster with enough VRAM capacity but more limited computational power. However, the key takeaway is that we are able to train two models that we were not able to train before using the full fine-tuning approach.
It’s also important to note that the same behavior is observed in the LoRA experiments with the latest RHOAI version, demonstrating increasing performance compared to the baseline RHOAI 2.16.

Even though LoRA can underperform full fine-tuning in terms of training runtime and throughput in compute-bounded scenarios, it will always present a smaller memory footprint, mainly due to the fact that only the LoRA adapter weights are updated during training. In this case, the LoRA memory savings add up to more than a 50% reduction compared to the full fine-tuning equivalents (Figure 8).

The throughput analysis (Figure 9) reveals a pattern that mirrors our training runtime observations: in compute-bounded scenarios, LoRA’s throughput can lag behind full fine-tuning. This demonstrated characteristic reinforces the computational trade-off inherent in LoRA’s inner working. While excelling at memory efficiency, it adds computational complexity that becomes evident when computational power is the primary bottleneck.

The third testing setup
This third scenario focuses on fine-tuning using the QLoRA algorithm and squeezing every drop of performance from your bits and bytes. The algorithm resembles LoRA, but operates on a quantized model. This quantization can occur either before fine-tuning begins or on-the-fly during the fine-tuning process itself. Technically, QLoRA fine-tuning should consume less GPU memory compared to its LoRA equivalent but it’s expected to take longer to run, since parameter updates still happen in full precision. So extra computational time is spent quantizing and de-quantizing the parameters to perform such updates.
This test consists of running QLoRA fine tuning for a given set of models in an OpenShift cluster. The underlying LoRA algorithm uses a rank (r) of 4 and a scaling factor (alpha) of 16. The test settings are as follows:
- Dataset: Cleaned Alpaca Dataset
- Replication factor of the dataset (where 1.0 represents full dataset): 0.2
- Number of accelerators: 4xH100-80GB NVIDIA GPU
- Maximum sequence length: 512
- Epochs: 1
In this particular case, we used GPTQ-LoRA, which is a method that expects a quantized base model. For the quantization, the 4-bit AutoGPTQ technique was used.
Fine-tuned models:
- mistral-7b-v0.3-gptq
- granite-8b-code-instruct-gptq
- allam-beta-13b-chat-gptq
- granite-34b-code-base-gptq
- mixtral-8x7b-instruct-v0.1-gptq
In this case, we used different models since we had access to the pre-quantized model. However, it’s worth mentioning that thanks to our automation that makes full use of RHOAI capabilities, it is remarkably straightforward to configure and deploy new experiments with varied settings and models.
Even though we did not have access to quantized versions of our previous tested models, we can still draw conclusions from the training runtime results shown in Figure 10. For instance, the model mixtral-8x7b-instruct-v0.1-gptq takes around 12754 seconds to finish its training loop while the model mistralai/Mixtral-8x7B-Instruct-v0.1 from our LoRA example takes around 1907 seconds, which is around 7 times less training runtime. But don’t worry, as mentioned, this is fully expected due to the nature of QLoRA and the multiple quantizations and dequantizations that happen in each pass.

QLoRA truly distinguishes itself in GPU memory efficiency, significantly reducing the consumption of VRAM (Figure 11). Following the previous example, the model mixtral-8x7b-instruct-v0.1-gptq uses merely 70Gi to complete its training cycle while the model mistralai/Mixtral-8x7B-Instruct-v0.1 from our LoRA experiment needs a substantial 153Gi, more than double the memory footprint. The memory savings stem directly from QLoRA's quantization techniques that maintain gradient flow precision while drastically reducing the memory requirements for model weights.

As we have seen before, throughput is naturally interrelated to training runtime, so it is no surprise that QLoRA’s throughput is low compared to the LoRA implementation (Figure 12). What stands out prominently in the data, however, is the consistent performance advantage demonstrated by the latest RHOAI version over its predecessors across all testing scenarios.
The fully automated pipeline not only ensured methodological consistency across test iterations but also enabled the precise performance differentials that have been documented between successive RHOAI versions, performance gains or regressions that might otherwise be obscured by inconsistencies in manual testing procedures.

Future work
Our explorations of LLM fine-tuning approaches on Red Hat OpenShift AI (RHOAI) provide significant insights into performance characteristics and methodological trade-offs, yet this represents merely the initial phase of deeper technical investigations that took place along the way. Several research lines have emerged during our testing and we will include all of those findings in future articles.
Some of those findings have been synthesized for the sake of this article, but we will expand. One of the main sets of experiments that we ran for this work lay around LoRA and full fine-tuning performance comparison in both memory-bounded and compute-bounded scenarios.
Similarly, with the performance and scalability validation framework now solidly established, our experiment's focus will also include the qualitative implications of these different fine-tuning methodologies. It’s very interesting to examine how the technical trade-offs we have documented here translate into practical model capabilities.
The quantitative foundations established here provide the necessary context for these qualitative investigations, enabling meaningful comparisons between approaches beyond simple efficiency metrics. Stay tuned for the next installment of our series.